Browse our range of reports and publications including performance and financial statement audit reports, assurance review reports, information reports and annual reports.
Governance of Artificial Intelligence at the Australian Taxation Office
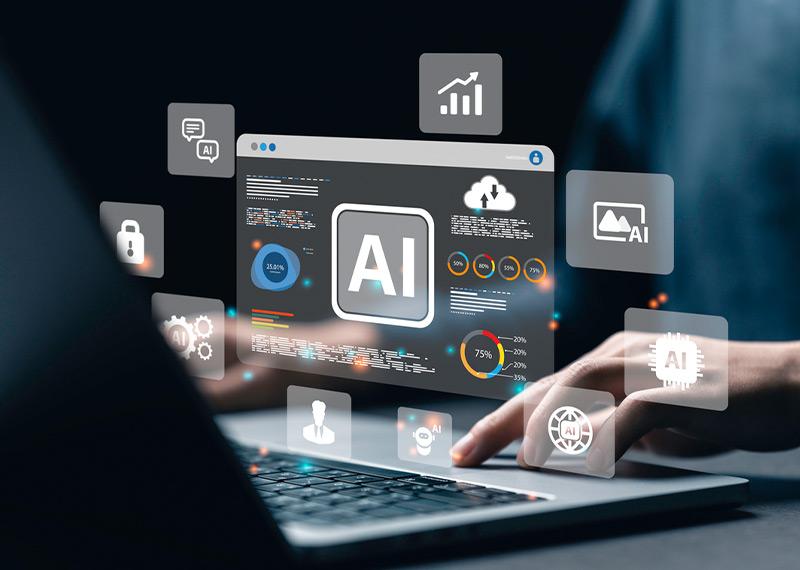
Please direct enquiries through our contact page.
Audit snapshot
Why did we do this audit?
- The term artificial intelligence (AI) incorporates a range of technologies. AI technologies are evolving and the use of AI is expanding.
- AI has the potential to support public sector entities to improve productivity, services and their effectiveness. On the other hand, there are risks that need to be managed.
- The Australian Taxation Office (ATO) uses AI, and it has plans to expand its use.
- This audit provides assurance to the Parliament over whether the ATO has effective arrangements to support the adoption of AI.
Key facts
- The Australian Government Policy for the responsible use of AI in government took effect from 1 September 2024.
- AI is used in different contexts by the ATO, including to: analyse data to assess non-compliance risks; assist with the drafting of communications; and help with visualisations.
What did we find?
- The ATO has partly effective arrangements in place to support the adoption of AI, including arrangements for: governance; design, development and deployment; and monitoring, evaluation and reporting.
- The ATO is adapting its current arrangements and introducing new arrangements to support its adoption of AI.
What did we recommend?
- The ANAO made seven recommendations to the ATO relating to AI governance, risk management, evaluation and information management.
- The ATO agreed to all seven recommendations.
43
ATO-built AI models in production at the ATO, as of 14 May 2024.
8
Number of publicly available generative AI tools approved as low risk by the ATO, as of 18 June 2024.
74%
Percentage of the ATO’s AI models in production that did not have completed data ethics assessments, as of August 2024.
Auditor-General’s foreword
Emerging technologies including artificial intelligence (AI) are increasingly a part of public services, with 56 public sector entities advising in the Australian National Audit Office (ANAO)’s 2023–24 financial statements audits that they have adopted AI in their operations. AI can offer the promise of better services, enhanced productivity and efficiency — and also has the potential for increased risk and unintended consequences.
AI is an area of public interest for the Australian Parliament, with two inquiries underway during the time of undertaking this audit. The Select Committee on Adopting AI reported in November 2024.1 At the time of presenting this audit report to the Parliament, the Joint Committee of Public Accounts and Audit is conducting an inquiry into the use and governance of AI systems by public sector entities.2 The Australian Government has policies and frameworks for agencies on the adoption and use of AI that are referred to in this audit.
The growing use of AI also brings new challenges and opportunities in auditing. As a first step in addressing these, the ANAO has identified providing assurance on the governance of the use of new technology as a way of bringing transparency and accountability to the Parliament in this area of emerging public administration. The Australian Taxation Office (ATO), as an agency that uses technology extensively in its administration of the tax and superannuation systems, was chosen as the first agency in this new line of audit work. I acknowledge the ATO’s work on governance to support rapidly emerging technologies and cooperation in the undertaking of this audit. I also acknowledge the assistance of the Digital Transformation Agency through consultation on this audit.
The ANAO will continue to focus on governance of AI while it develops the capability to undertake more technical auditing of the AI tools and processes used in the public sector. Building this capability will require investment in knowledge, methodology and skills to enable the ANAO to test more deeply how AI tools operate in practice.
Like audit offices around the world, the ANAO will seek to examine how AI can improve the audit process itself, in a profession where human judgement and scepticism are foundations in auditing standards. This work will progress through our relationships within the international public sector audit community over coming years.
Dr Caralee McLiesh PSM
Auditor-General
Summary and recommendations
Background
1. The term artificial intelligence (AI) encompasses a broad range of technologies, some of which have been in existence for many years. The Australian Government (the government) has adopted the Organisation for Economic Co-operation and Development (OECD’s) definition of an AI system.
An AI system is a machine-based system that for explicit or implicit objectives, infers, from the input it receives, how to generate outputs such as predictions, content, recommendations, or decisions that can influence physical or virtual environments. Different AI systems vary in their levels of autonomy and adaptiveness after deployment.3
2. There is no one-size-fits-all governance approach to support the adoption of AI. At the entity level, governance and assurance arrangements should be commensurate with the level of risk. Existing governance arrangements may need to be adapted or updated for AI, or new ones may need to be established.
3. The government has committed to adopting AI to ‘improve user experience, support evidence-based decisions and gain efficiencies in agency operations’ and to equip entities to safely engage with emerging technologies, including AI.4 In 2024, the government released an assurance framework for the use of AI in government5 and a policy on the responsible use of AI6, with an aim of positioning the government as an exemplar in the safe and responsible use of AI.
4. The Australian Taxation Office (ATO) uses AI in a variety of contexts and has committed to the ethical and lawful adoption of AI. It plans to expand its use of AI over the coming years. The ATO’s primary use of AI involves AI models that it has built in house7, and publicly available generative AI tools that is has assessed as low-risk and approved for use. As of 14 May 2024, the ATO had 43 AI models in production and, as of 18 June 2024, eight publicly available generative AI tools approved for use. Table 2.1 provides further information about the ATO’s use of AI.
Rationale for undertaking the audit
5. AI technologies offer organisations, including public sector entities, a range of opportunities. AI can help public sector entities to drive productivity growth, to improve service delivery and to more effectively deliver on their purposes. On the other hand, issues that need to be managed include the risk of bias, lack of transparency and accountability, privacy, security and legality. This audit provides independent assurance to the Parliament as to whether the ATO has effective arrangements in place supporting its adoption of AI.
Audit objective and criteria
6. The objective of the audit was to assess whether the ATO has effective arrangements in place to support the adoption of AI.
7. To form a conclusion against the objective, the following criteria were adopted.
- Does the ATO have effective governance arrangements supporting the adoption of AI?
- Has the ATO established effective arrangements to support the design, development and deployment of AI models?
- Is the ATO effectively monitoring, evaluating and reporting on the adoption of AI?
Conclusion
8. The ATO has partly effective arrangements in place to support its adoption of AI. Within the Australian Government sector, requirements and guidance for AI governance and management are evolving. In 2024, there were a range of AI-related initiatives underway, including that the government policy on the responsible use of AI in government took effect from September 2024. The ATO is adapting existing data management and data governance arrangements and introducing new arrangements to support its adoption of AI, including to support risk management and assessments of ethical considerations. It lacks effective arrangements for the design, development, deployment and monitoring of its AI models.
9. The ATO has partly effective governance arrangements supporting its adoption of AI. In December 2023, it introduced a policy on the use of publicly available generative AI tools and it developed an automation and AI strategy in October 2022. The ATO has not: established fit-for-purpose implementation arrangements for this strategy; clearly defined enterprise-wide roles and responsibilities; established AI-specific risk management arrangements; and implemented its data ethics framework sufficiently for AI.
10. The ATO has partly effective arrangements supporting the design, development and deployment of its AI models.
- The ATO does not have specific policies and procedures for the design, development and deployment of its AI models, although there are enterprise policies and procedures which are relevant. The lack of approved and embedded policies and procedures creates risks to the effective implementation of models.
- The ATO has not sufficiently integrated ethical and legal considerations into its design and development of AI models. This impairs the ability of the ATO to demonstrate that its AI models are: fair and free from bias; reliable and safe; protecting privacy; transparent and explainable; contestable; and have appropriate accountability arrangements.
- There are no clearly defined assurance and approval arrangements that set out testing, validation, review and decision-making throughout the design, development and deployment of AI models.
11. The ATO has partly effective arrangements to monitor, evaluate and report on its adoption of AI.
- There was no evidence of structured and regular monitoring of ATO-built AI models in production. This was being addressed through the development of a monitoring and reporting framework for its AI models.
- The ATO did not regularly report on the implementation of its automation and AI strategy between October 2022 and January 2024. It has developed a monthly report since February 2024 to report on the implementation of the strategy. It has not set out an evaluation approach for the strategy.
Supporting findings
Governance arrangements supporting the adoption of artificial intelligence
12. The ATO is developing a strategic framework to support its adoption of AI. It:
- is developing an AI policy and risk management guidance (due December 2025);
- has established a policy on the use of publicly available generative AI by ATO officers;
- does not have sufficient centralised visibility and oversight of its use of AI, impacting its ability to effectively govern the use of AI across the organisation; and
- has not established fit-for-purpose implementation arrangements for its automation and AI strategy. (See paragraphs 2.3 to 2.30)
13. The ATO uses existing organisational and governance structures to support its adoption of AI, and it has been adapting these for AI. Over time, the ATO has also established AI-focussed governance bodies. Key roles and responsibilities with respect to AI are not always clearly defined, including enterprise-wide responsibilities and accountabilities over the ATO’s AI governance framework and for AI models and systems. In September 2024, the ATO established a Data and Analytics Governance Committee in recognition that stronger governance arrangements were needed. In November 2024, the ATO appointed its Chief Data Officer as its accountable official under the Policy for the responsible use of AI in government. (See paragraphs 2.31 to 2.45)
14. There are risks related to the adoption of AI at various levels at the ATO.
- The ATO has two enterprise risks that relate to AI due to their focus on data and analytics. These risks are ‘above tolerance’.
- The ATO has risk assessment processes that apply to its adoption of AI. It has identified that these are not sufficient for AI-specific risks, and it is working to introduce processes that better support the management of AI risks.
- The ATO has a risk-based approach to approving the use of publicly available generative AI technologies by ATO officers. (See paragraphs 2.46 to 2.74)
15. The ATO’s data ethics framework aims to support the ATO to deliver ethical data activities, including AI. For its AI models, the ATO has not complied with the requirements of this framework (74 per cent of AI models in production did not have completed data ethics assessments). This undermines the ATO’s ability to deliver and to assure the delivery of AI that aligns with ethical principles. The ATO has not developed effective monitoring and assurance arrangements for its data ethics framework. (See paragraphs 2.75 to 2.93)
Arrangements supporting the design, development and deployment of artificial intelligence models
16. The ATO has not established a framework of policies and procedures for the design of AI models. For the 14 models that the ATO developed and deployed between 1 July 2023 and 14 May 2024, there were mixed practices related to planning, governance and design. The ATO largely defined business problems to be solved by AI models, defined roles and responsibilities and documented stakeholder engagement. There were gaps in terms of: project planning and risk management; assessment of ethical, security, privacy and legal considerations; and assurance, decision-making and record keeping. For the work-related expenses AI models8, there were gaps in how the ATO assessed ethical, privacy and legal considerations. (See paragraphs 3.2 to 3.16)
17. The ATO has not established a framework of policies and procedures for the development of AI models, although model development is to be documented in a standardised modelling solution report. For the 14 models that the ATO deployed between 1 July 2023 and 14 May 2024, there were differences in approaches as to: how data suitability was assessed and documented within the context of each model; how testing and validation was conducted; and decision-making arrangements for the development phase. For the work-related expenses AI models, the ATO assessed two potential biases. There was a lack of evidence to demonstrate that the ATO had considered whether data was fit for purpose and documented considerations relating to reproducibility. (See paragraphs 3.17 to 3.25)
18. The ATO’s IT change enablement policy applies to all IT changes at the ATO, including the deployment of its AI models. For the 14 models that the ATO deployed between 1 July 2023 and 14 May 2024, practices varied for defining deployment criteria and deploying models. The ATO planned for model deployment and partially conducted verification and validation of models. (See paragraphs 3.26 to 3.31)
Monitoring, evaluating and reporting on the adoption of artificial intelligence
19. The ATO does not have policies and procedures supporting the monitoring and evaluation of its in-house built AI models. For the 14 AI models built and deployed between 1 July 2023 and 14 May 2024, there was no evidence of ongoing performance monitoring and reporting. For the work-related expenses AI models, there were some examples of monitoring and evaluation. Baselines were not reported in ongoing monitoring and reporting to show the impact of introducing the models. (See paragraphs 4.3 to 4.9)
20. The ATO has a project underway to introduce an enterprise-wide approach to monitoring the performance of its AI models by December 2026. The ATO has developed a ‘use of publicly available generative AI technology policy’. The ATO does not report on compliance with this policy to relevant internal governance bodies. For its automation and AI strategy, the ATO introduced status reporting in February 2024. It does not have arrangements in place to measure the effectiveness of the strategy. Some continuous improvement arrangements were evident including an internal governance review and the delivery of the automation and AI strategy. There is a need for the ATO to improve its management of information in support of the transparent and accountable adoption of AI. (See paragraphs 4.10 to 4.32)
Recommendations
Recommendation no. 1
Paragraph 2.29
The ATO align implementation arrangements for the automation and AI strategy with enterprise-wide requirements.
Australian Taxation Office response: Agreed.
Recommendation no. 2
Paragraph 2.44
The ATO clearly define and communicate enterprise-wide organisational structures and governance arrangements supporting its adoption of AI, including defining accountabilities and responsibilities at the model and system level.
Australian Taxation Office response: Agreed.
Recommendation no. 3
Paragraph 2.61
The ATO review the misuse of data and analytics enterprise risk in accordance with its enterprise risk management framework and risk appetite, and update and incorporate controls relating to the impact of AI on this risk.
Australian Taxation Office response: Agreed.
Recommendation no. 4
Paragraph 2.92
The ATO improve its arrangements in support of the design, development, deployment and use of AI that aligns with ethical principles by:
- specifying requirements relating to AI reproducibility and auditability;
- ensuring the data ethics framework is integrated into other ATO processes;
- completing ethics assessments for the AI models in production; and
- introducing monitoring, assurance and reporting arrangements over the implementation of its data ethics framework.
Australian Taxation Office response: Agreed.
Recommendation no. 5
Paragraph 3.30
The ATO develop and implement policies and procedures to support the effective design, development, deployment and assurance of AI models. Where relevant ATO policies and procedures exist, the ATO ensure that the design, development and deployment of AI models aligns with these.
Australian Taxation Office response: Agreed.
Recommendation no. 6
Paragraph 4.22
The ATO establish performance measurement and evaluation arrangements for its automation and AI strategy.
Australian Taxation Office response: Agreed.
Recommendation no. 7
Paragraph 4.31
The ATO ensure that its approach to managing information supports transparency and accountability with respect to its adoption of AI.
Australian Taxation Office response: Agreed.
Summary of entity response
21. The proposed audit report was provided to ATO. The ATO’s summary response is provided below, and its full response is included at Appendix 1. Improvements observed by the ANAO during the course of this audit are listed in Appendix 2.
The ATO aims to continually improve its use of data and analytics to derive the insights needed to give better clarity and certainty for our decisions and actions. The ATO remains committed to managing taxpayer data with integrity and ensuring ethical decision making in everything we do. We recognise the importance of robust governance and accountability to support the development and use of analytical models that are ethical, safe and deliver outcomes that are fit for purpose.
The ATO therefore welcomes this review and the ANAO’s insights as to how to continue to improve our artificial intelligence (AI) related governance. We also have appreciated the opportunity to assist the ANAO benchmark its approach to conducting similar AI use and governance audits in the future, as well as providing insights to other APS Agencies.
We are proud to be a leading agency of data governance and management in the APS. We acknowledge what is considered leading practice for AI use and governance is still evolving and we will continue to not only strive to achieve leading practice, but also assist the broader APS. The next phase of maturing our data governance has commenced as we develop and implement AI specific policies and guidance.
We agree with the seven recommendations in the report and are working to implement them. The recommendations will help us evolve our existing governance and practices to remain current in the face of rapidly advancing AI capability.
Key messages from this audit for all Australian Government entities
22. Below is a summary of key messages, including instances of good practice, which have been identified in this audit and may be relevant for the operations of other Australian Government entities.
Governance and risk management
Performance and impact measurement
Record keeping
1. Background
Introduction
1.1 Technologies considered to be artificial intelligence (AI) have been in existence for many years. AI has the potential to support public sector entities to drive efficiencies and productivity growth, to improve service delivery and to deliver on their purposes more effectively. On the other hand, poor implementation of AI in public service delivery could risk eroding trust in the public service or cause harm.9
1.2 Good governance and assurance arrangements support the delivery of ethical and lawful AI.10 Given the breadth of AI, the Organisation for Economic Co-operation and Development (OECD) has highlighted that there is no one-size-fits-all approach to AI governance:
different AI systems bring different benefits and risks. In comparing virtual assistants, self-driving vehicles and video recommendations for children, it is easy to see that the benefits and risks of each are very different. Their specificities will require different approaches to policy making and governance.11
1.3 The Australian Taxation Office (ATO) employs AI in a variety of contexts to analyse large datasets for the provision of analysis and assessments.12 The ATO’s primary use of AI involves AI models that it has built in house, and publicly available generative AI tools that it has assessed as low-risk and approved for use. As of 14 May 2024, the ATO had 43 AI models in production and, as of 18 June 2024, eight publicly available generative AI tools approved for use. Table 2.1 provides further information about the ATO’s use of AI.
Artificial intelligence
1.4 There is no single commonly agreed upon definition of AI. The OECD has identified that this may create challenges when developing legislation and regulation for AI.13 As technology has advanced and evolved, definitions have changed and may continue to change.14
1.5 The Australian Government (the government) has adopted the OECD’s definition of an AI system and suggests that entities should keep up to date on changes to this definition (Box 1).15
Box 1: Definition of an AI system |
In November 2023, OECD countries adopted a consensus definition of an AI system:
|
Note a: OECD, Explanatory memorandum on the updated OECD definition of an AI system, OECD, March 2024, p. 4, available from https://www.oecd-ilibrary.org/science-and-technology/explanatory-memorandum-on-the-updated-oecd-definition-of-an-ai-system_623da898-en [accessed 5 April 2024].
1.6 AI systems can be broadly categorised as:
- narrow or weak AI — an AI system that is ‘trained’ to deliver outputs for specific tasks to address specific problems, such as search engines or facial recognition; or
- general purpose or strong AI — an AI system that can be used for a broad range of tasks, both intended and unintended by developers, such as text or image generation.16
Artificial intelligence in the Australian Government sector
1.7 The government has stated that AI has the potential to enhance Australia’s wellbeing, quality of life and economic growth.17 In its Data and Digital Government Strategy published in December 2023, the government committed to adopting AI to ‘improve user experience, support evidence-based decisions and gain efficiencies in agency operations’ and to equip entities to safely engage with emerging technologies, including AI.18
OECD AI Principles and Australia’s AI Ethics Framework
1.8 The ethical use of AI involves promoting the responsible design, development and implementation of AI that is ‘innovative and trustworthy and that respects human rights and democratic values’.19 Ethical AI practices assist organisations to implement AI technologies that are safer, more reliable and fairer, and reduce the risks of negative outcomes.20
1.9 The OECD has developed five AI principles around: inclusive growth, sustainable development and wellbeing; human rights and democratic values, including fairness and privacy; transparency and explainability; robustness, security and safety; and accountability.21
1.10 The government committed to meeting the OECD principles through Australia’s AI Ethics Framework which was published in November 2019.22 This framework ‘guides businesses and governments to responsibly design, develop and implement AI’ and includes eight AI Ethics Principles (see Figure 1.1).23 The framework states that by applying the ethics principles and committing to ethical AI practices, organisations can build public trust, positively influence outcomes from AI, and ensure all Australians benefit from this technology.
Figure 1.1: Australia’s AI Ethics Principles
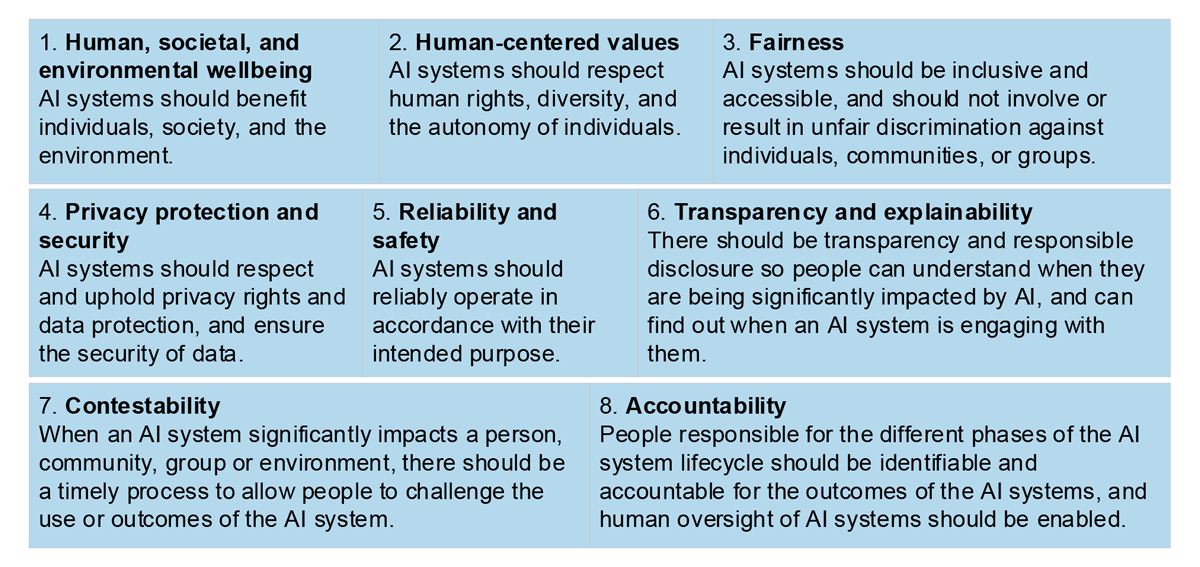
Source: Department of Industry, Science and Resources, Australia’s AI Ethics Principles.
Use and initiatives
1.11 As of 30 June 2024, 56 Australian Government entities had reported to the ANAO that they had adopted AI (2022–23: 27 entities).24 Most of these entities had adopted AI for research and development activities, IT systems administration and data and reporting. Of the 56 entities adopting AI, 36 (64 per cent) established internal policies specifically governing the use of AI, while 15 (27 per cent) established internal policies regarding assurance over AI use. Use of AI by entities includes: chatbots, virtual assistants and agents in service management; document and image recognition; to support law enforcement; data mapping to geographical areas; and, as noted in Table 1.1, a trial of Copilot for Microsoft 365.
1.12 Table 1.1 presents a timeline of key Australian Government and parliamentary initiatives relating to AI between 1 July 2023 and 31 December 2024.
Table 1.1: Key Australian Government and parliamentary AI initiatives, July 2023 to December 2024
Date |
Initiative |
6 July 2023 |
The Digital Transformation Agency released ‘Interim guidance on generative AI for Government agencies’. An update was published on 22 November 2023. |
20 September 2023 |
The AI in Government Taskforce was established to develop whole-of-government AI policies, standards and guidance. It comprised secondees from 11 entities, including the ATO. It ended in June 2024. |
13 November 2023 |
The government responded to the Royal Commission into the Robodebt Scheme. In response to recommendation 17.2 (establishment of a body to monitor and audit automated decision-making), the government stated that it would ‘ensure there is appropriate oversight of the use of automation in service delivery’. It noted that this would include AI. |
15 December 2023 |
|
1 January – 30 June 2024 |
More than 50 Australian Public Service entities, including the ATO, undertook a six-month trial of Copilot for Microsoft 365.a |
2 February 2024 |
The AI Expert Group was established to advise on proposed mandatory guardrails for the safe design, development and deployment of AI systems in high-risk settings. The group includes government and non-government members. |
21 June 2024 |
The National framework for the assurance of artificial intelligence in government was released to set ‘foundations for a nationally consistent approach to AI assurance’. |
15 August 2024 |
The government released the Policy for the responsible use of AI in government with effect from 1 September 2024 to position the government as an exemplar under its safe and responsible AI agenda. It includes mandatory requirements for accountable officials by 30 November 2024 and transparency statements by 28 February 2025. |
September 2024 |
The Digital Transformation Agency commenced the pilot of the Australian Government AI Assurance Framework with a group of entities, including the ATO. |
5 September 2024 |
The government announced further consultation on guardrails for AI in high-risk settings and released the Voluntary AI Safety Standard. |
12 September 2024 |
The Joint Committee of Public Accounts and Audit commenced an inquiry into the use and governance of AI systems by public sector entities. |
11 October 2024 |
The Senate Select Committee on Adopting Artificial Intelligence released its interim report, making five recommendations. It was established in March 2024 to inquire into and report on the opportunities and impacts arising out of the uptake of AI. |
11 October 2024 |
The Digital Transformation Agency released Guidance for staff training on AI and an AI in government fundamentals training module. |
23 October 2024 |
The Digital Transformation Agency released an evaluation of the whole-of-government trial of Copilot for Microsoft 365. The evaluation concluded that ‘there are clear benefits to the adoption of generative AI but also challenges with adoption and concerns that need to be monitored’. |
26 November 2024 |
The Senate Select Committee on Adopting Artificial Intelligence tabled its final report. It made 13 recommendations. |
13 December 2024 |
The government announced that it would develop a National AI Capability Plan to support growth of Australia’s AI capabilities. |
17 December 2024 |
The government released a whole-of-government data ethics framework to provide guidance on best practice for ethical considerations relating to public data use. |
Note a: Copilot for Microsoft 365 is a generative artificial intelligence chatbot/assistant which integrates with Microsoft 365 products. The ANAO participated in this trial.
Source: ANAO analysis.
National framework for the assurance of artificial intelligence in government
1.13 In June 2024, the Australian Government and state and territory governments released the National framework for the assurance of artificial intelligence in government to support Australia’s governments in ‘gaining public confidence and trust in the safe and responsible use of AI’.25 The framework is based on Australia’s AI Ethics Principles with the intention to establish a ‘nationally consistent’ approach to foundations for AI assurance practices across all aspects of government.26
Policy for the responsible use of AI in government
1.14 In August 2024, the government released the Policy for the responsible use of AI in government to position the Australian Government sector ‘as an exemplar under its broader safe and responsible AI agenda’ and ‘to create a coordinated approach to the government’s use of AI’.27 The policy came into effect on 1 September 2024 and requires28 that:
- entities designate ‘accountable officials’ by 30 November 202429; and
- entities publish AI transparency statements by 28 February 2025 and update these annually or sooner, if they make significant changes to their approach to AI.30
Artificial intelligence at the Australian Taxation Office
1.15 The ATO’s purpose is ‘to contribute to the economic and social wellbeing of Australians by fostering willing participation in the tax, superannuation, and registry systems’.31 The ATO states that it uses data and analytics (including AI) to: understand and improve interactions with its ‘clients’; make better, faster and smarter decisions; deliver outcomes with ‘agility’; and support advice to government.32
1.16 The ATO has stated that its data activities (including its use of AI) must be both lawful and ethical. The ATO’s six data ethics principles (see paragraphs 2.77 to 2.79) set the minimum standards that must be considered when collecting, using, sharing, archiving, and disposing of data. Through these principles, the ATO aims to address the main identified ethical risks that may arise in data activities and ensure that data is used by the ATO in an appropriate way.
1.17 The ATO reported on the way that it uses AI:
The ATO uses AI tools to increase the efficiency and effectiveness of work done by staff enabling us to deliver better services and greater value to the community. The ATO currently uses AI to review large quantities of unstructured data for risk and intelligence purposes, power risk models to identify potential non-compliance for human review, and draft and edit communications. The ATO has human oversight over all uses of AI, and decision making that impacts clients is always made by a human.33
1.18 The ATO uses AI in a variety of contexts including: to assess risks associated with submitted claims, such as individual tax returns; to help manage its call centre volumes; and to provide its virtual assistant on the ATO website, Alex (see Table 2.1 for an overview of AI in use at the ATO).
1.19 The ATO has participated in government AI-related initiatives, such as: the AI in Government Taskforce; the Copilot for Microsoft 365 trial; the trial of the Australian Government AI assurance framework; and the development of a whole-of-government Data Ethics Framework.
Previous audits and reviews
1.20 In Audits of the Financial Statements of Australian Government Entities for the Period Ended 30 June 2024, the ANAO outlined that an ‘absence of frameworks governing the use of emerging technologies could increase the risk of unintended consequences’. The ANAO reported that during 2023–24, ‘64 per cent of entities that used AI had also established internal policies governing the use of AI (2022–23: 44 per cent). Twenty-seven per cent of entities had established internal policies regarding assurance over AI use’.34
Rationale for undertaking the audit
1.21 AI technologies offer organisations, including public sector entities, a range of opportunities. AI can help public sector entities to drive productivity growth, to improve service delivery and to more effectively deliver on their purposes. On the other hand, issues that need to be managed include the risk of bias, lack of transparency and accountability, privacy, security and legality. This audit provides independent assurance to the Parliament as to whether the ATO has effective arrangements in place supporting its adoption of AI.
Audit approach
Audit objective, criteria and scope
1.22 The objective of the audit was to assess whether the ATO has effective arrangements in place to support the adoption of AI.
1.23 To form a conclusion against the objective, the following criteria were adopted.
- Does the ATO have effective governance arrangements supporting the adoption of AI?
- Has the ATO established effective arrangements to support the design, development and deployment of AI models?
- Is the ATO effectively monitoring, evaluating and reporting on the adoption of AI?
1.24 The audit scope included:
- an examination of the ATO’s governance arrangements applying to its adoption of AI, with a focus on the period from 1 July 2022 to 30 June 2024;
- an examination of the design, development, deployment and monitoring of 14 AI models deployed by the ATO between 1 July 2023 and 14 May 2024 — see Appendix 3 for a list and description of these models; and
- an examination of the ATO’s AI models that it uses in the context of processing and assessing work-related expenses claims — see Appendix 4 for a list and description of these models.
1.25 The audit scope does not include an examination of the ATO’s data governance and data management more broadly.
Audit methodology
1.26 The audit methodology involved:
- examination of entity records, including email records and electronic documentation;
- meetings with ATO officers and external stakeholders;
- walkthroughs of ATO systems and analysis of selected AI models; and
- review of citizen contributions to the audit.
1.27 Appendix 5 provides an overview of the sources (legislation, standards, policies and guidance) that have informed the methodology for this audit.
1.28 The audit was conducted in accordance with ANAO Auditing Standards at a cost to the ANAO of approximately $892,944.
1.29 The team members for this audit were Nathan Callaway, Dr Shannon Clark, Stewart Hafey, Kayla Hurley, Nancy Jin, Kelvin Le, Zhuo Li, Alyssa McDonald, Benjamin Siddans and David Tellis.
2. Governance arrangements supporting the adoption of artificial intelligence
Areas examined
This chapter examines whether the Australian Taxation Office (ATO) has effective governance arrangements in place supporting its adoption of artificial intelligence (AI).
Conclusion
The ATO has partly effective governance arrangements supporting its adoption of AI. In December 2023, it introduced a policy on the use of publicly available generative AI tools and it developed an automation and AI strategy in October 2022. The ATO has not: established fit-for-purpose implementation arrangements for this strategy; clearly defined enterprise-wide roles and responsibilities; established AI-specific risk management arrangements; and implemented its data ethics framework sufficiently for AI.
Areas for improvement
The ANAO made four recommendations aimed at: establishing implementation arrangements for the ATO’s automation and AI strategy; defining roles and responsibilities; reviewing the ATO’s misuse of data and analytics risk; and enhancing the arrangements supporting the delivery of AI that aligns with ethical principles.
The ANAO also suggested that the ATO could: update relevant policies and procedures; have a register of all AI; and improve risk management arrangements for generative AI.
2.1 AI governance is an evolving area, with a common theme that there is no one-size-fits-all governance model. The National framework for the assurance of artificial intelligence in government outlines that ‘[g]overnance structures should be proportionate and adaptable to encourage innovation while maintaining ethical standards and protecting public interests’.35
2.2 At the organisational level, AI governance includes: establishing an AI policy and strategy; assigning and communicating roles and responsibilities; establishing risk management arrangements; and integrating ethical considerations into the design and development of AI systems.
Does the ATO have an effective strategic framework supporting the adoption of artificial intelligence?
The ATO is developing a strategic framework to support its adoption of AI. It
- is developing an AI policy and risk management guidance (due December 2025);
- has a policy on the use of publicly available generative AI by ATO officers;
- does not have sufficient centralised visibility and oversight of its use of AI, impacting its ability to effectively govern the use of AI across the organisation; and
- has not established fit-for-purpose implementation arrangements for its automation and AI strategy.
Artificial intelligence policy
2.3 The ATO’s data management and data governance policies apply to its use of AI.36 The ATO’s Data Management Chief Executive Instruction (data management policy) sets out requirements for managing data throughout the data lifecycle.37 The ATO states that its data governance arrangements incorporate: policy, standards and guidance; organisational structures and oversight mechanisms; culture, ethics and behaviour; people, skills and competencies; and compliance and issues management.
2.4 While the ATO’s data management and data governance arrangements apply to AI, it has identified that these are not fit for purpose for AI. This includes that: existing arrangements do not sufficiently capture AI-specific risks; there is no defined process when undertaking AI-related activities; there are staff capability gaps around AI-specific risks and data management obligations; there is minimal AI-specific governance, with limited enterprise visibility; and there are few controls to manage risks associated with AI activities.
2.5 The ATO was updating or had plans to update several aspects of its data management and data governance arrangements for AI.
- The ATO was developing an AI policy and AI risk management guidance. These were expected to be delivered in 2024, however, as of December 2024, are expected by December 2025. The ATO has identified that a ‘complex consultation process is required to stand up’ the new policy.38
- The ATO’s data management policy mandates the application of the ATO’s data ethics framework and data stewardship model for all data activities, including AI. In August 2024, the ATO updated its ethics assessment processes for analytical models, including AI models (see paragraph 2.81).
2.6 As part of an April 2024 internal review by the ATO, the ATO sought to determine the extent to which AI requires its own governance and management arrangements. The conclusion was that ‘AI requires a more distinct approach since specialist knowledge is required to be able to manage the unique issues and risks that AI poses’. In the review, the ATO assessed that its framework39 for AI had the most significant gaps (compared to data, analytics and automation frameworks) and the lowest level of maturity.40 It also noted a lack of a strategy to fill the gaps at the time. The ATO advised the ANAO on 16 September 2024 that ‘prioritisation and resource constraints have slowed planned work to address data governance gaps’.
2.7 The Policy for the responsible use of AI in government outlines that entities should integrate AI considerations into existing frameworks such as those for privacy, protective security, record keeping, cyber and data.41 As of July 2024, the ATO had not reviewed other relevant ATO frameworks and policies to assess their applicability to AI and to determine if they need to be updated and adapted for AI.
Opportunity for improvement
2.8 The ATO could review and update other enterprise policies and procedures for AI, as appropriate.
Use of publicly available generative AI policy
2.9 The Australian Government released guidance on the use of publicly available generative AI tools in July 2023, with updated guidance in November 2023.42 In December 2023, the ATO finalised a policy for ATO officers on using publicly available generative AI technology.43 The policy sets out processes aimed at supporting the appropriate and responsible use of publicly available generative AI tools by ATO officers. The policy states that ‘publicly available generative AI technologies may be approved for ATO work purposes, on ATO devices and networked computers, where the risk of negative impact is low’. The ATO’s oversight of this policy is discussed at paragraph 2.39 and its approach to assessing the risk of publicly available generative AI technologies is discussed at paragraphs 2.69 to 2.73.
Artificial intelligence at the ATO
2.10 Prior to the release of the Policy for the responsible use of AI in government, the ATO defined AI as a ‘subset of computer science that deals with computer systems able to perform tasks normally requiring human intelligence, such as object recognition, speech recognition, decision-making and language translation’.
2.11 In October 2024, the ATO reported that it has adopted the Organisation for Economic Co-operation and Development (OECD) definition of AI in accordance with the Policy for the responsible use of AI in government. It has also stated that it ‘refers to any application of machine learning, deep learning and generative AI as AI. But does not consider rules-based analytics to be AI, as this form of analytics does not infer how to generate outputs from the inputs they receive’.44 Table 2.1 provides an overview of AI in use at the ATO.
Table 2.1: AI in use at the ATO
Category |
Description |
AI models built and developed by the ATO |
On 14 May 2024, the ATO provided the ANAO with a list of 43 modelsa in production that it defined as being AI. These models were developed in house by the ATO.
The ATO’s AI models use a range of machine learning algorithms, including natural language processing, deep learning and neural networks, in both supervised and unsupervised learning approaches. The ATO uses its AI models to support decision-making. None of the 43 models make fully automated decisions. Paragraph 2.15 sets out the nature of automated actions of these models. |
Publicly available generative AI |
The ATO has a register of publicly available generative AI technologies and associated uses approved for use under its ‘use of publicly available generative AI technology policy’. As of 18 June 2024, there were eight publicly available generative AI technologies approved for use within the ATO.c |
Other |
The ATO has some other uses of AI such as the virtual assistant on its website (Alex) and within call centres. |
Note a: The ATO defines AI models as: algorithms designed to mimic or surpass human intelligence and make predictions based on data; and that use mathematical, statistical or machine learning techniques trained on extensive datasets to process and analyse information.
The ATO’s AI models can link to one or more machine learning algorithms. The 43 AI models link to a total of 93 machine learning algorithms.
Note b: One of the work-related expenses AI models — document understanding — is not included in this list as it had not been put into production as of 14 May 2024.
Note c: These were: Microsoft Copilot; GitHub Copilot Visual Studio 2022 Extension for Business; Code Llama; Llama 2; Adobe Creative Cloud; OpenAI ChatGPT Team; IBM Cloud IaaS; and CoPilot for Microsoft 365.
Source: ANAO analysis of ATO documentation.
2.12 The ATO does not have a centralised inventory of all AI uses across the organisation. The ATO advised the ANAO on 16 September 2024 that there were no other uses of AI within the entity other than those listed in Table 2.1. Subsequent to this advice, in October 2024 the ATO reported to the Joint Committee of Public Accounts and Audit that it had also used AI to process 36 million documents to help identify entities of interest and their relationships, commencing in 2016. Although the original AI models used for this work have since been decommissioned, the ATO further advised the ANAO in January 2025 that it currently uses commercial software which incorporates AI for extracting intelligence from high-volume structured and unstructured data.45 This use is not included in Table 2.1.
Register and type of AI models
2.13 The National framework for the assurance of artificial intelligence in government (June 2024) states that an entity should maintain a register of when it uses AI, its purpose, intended uses and limitations.46
2.14 The ATO’s list of 43 AI models in production included the model name and grouping, and information about the timing of model execution. The list did not include information about each use case such as: the purpose and context of use; the type of model and technology infrastructure being used; the data used in both training and operation; ongoing cost; the results of recent ethical, privacy, legal and other risk assessments; performance information; and the extent of automation or automated decision-making.
2.15 Having oversight over the use of AI in relation to decision-making is important for governance and managing risks.47 The ATO advised the ANAO on 10 May 2024 and 22 July 2024 that of the 43 AI models, 30 did not include fully automated actions and 13 did.48 Eleven included automated ‘nudge’ messaging49; five included other automated messaging; seven included automated case selection for compliance action; and seven included other automated actions.50
2.16 Not having a centralised inventory of AI which includes key information impacts the ability of the ATO to effectively oversee and to be transparent and accountable with regard to its use of AI. Having good visibility and clearly defined purposes for AI models and systems is an important component of building fit-for-purpose governance. As at July 2024, the ATO was developing a register of its data and analytics models, including AI models. The ATO expects to finalise the register by March 2025.
Opportunity for improvement
2.17 The ATO could ensure that it has a complete and accurate inventory of all uses of AI across the organisation.
2.18 While the ATO does not maintain a register of key information about its AI models, the ATO’s 43 AI models could all be categorised as narrow AI (refer to paragraph 1.6) because they perform specific tasks or functions. The primary function of these models is to help the ATO analyse data in order to evaluate the risks that a taxpayer’s claims are not compliant, or to understand the impact tax agents have on their clients’ tax affairs.
Artificial intelligence strategy
Development and oversight of the strategy
2.19 The ATO commenced development of an automation and AI (A&AI) strategy in September 2020. The ATO did not have a planned approach to the development of this strategy. Consultation occurred across the ATO in developing the strategy — in the strategy, the ATO outlined that 17 business areas across the ATO were consulted to identify 52 potential A&AI use cases.
2.20 The ATO’s Data and Analytics Committee51 endorsed the A&AI strategy on 7 October 2022, two years after development commenced. In December 2022, the Data and Analytics Committee was dissolved, with its responsibilities transferred to the ATO’s Strategy Committee.52 The Strategy Committee makes decisions and provides advice in relation to the ATO’s strategies, oversees the implementation of strategies and monitors operational performance.
Goals of the strategy and linkages to other ATO strategies and plans
2.21 The ATO’s A&AI strategy states that there is a need for an ‘organisational strategy [for A&AI] to fully harness the benefits’. The vision is that ‘by 2030 the ATO is a leader in developing and industrialising ethical, impactful and scalable A&AI solutions, creating immense economic and social benefit for our nation and citizens’. Measurement against the strategy’s goals is discussed further in paragraphs 4.20 to 4.22.
2.22 The A&AI strategy has links to other ATO strategies and plans, including its corporate plan, digital strategy and data and analytics strategy. These strategies and plans incorporate strategic goals and objectives in relation to the adoption of AI.
2.23 Central to the ATO’s A&AI strategy is the delivery of five enterprise A&AI uses cases. The ATO outlined that use cases were prioritised based on value to deliver, feasibility of implementation and demand. The use cases aim to enhance existing or build new AI systems at the enterprise level. An overview of the five use cases is in Table 2.2.
Table 2.2: A&AI strategy use cases
Use case |
Objective |
Future state |
|
AI automatically reads, understands and analyses any document, saving significant human workload. |
Auto content extraction and understanding for most of the ATO’s common documents. |
|
Meet increasing need for real-time or pre-emptive insight and identification. |
Pre-emptive insight and action whenever possible. Real-time interaction whenever appropriate. |
|
Connect systems, data and intelligence to create a complete picture of taxpayers. |
Taxpayer enterprise-wide personalisation. Improved compliance and experience. Reduced management and compliance cost. |
|
AI learns from law frameworks and precedential rulings to suggest correct application of the law. |
Enterprise knowledge base that serves as a ‘second brain’: having all the knowledge, and capable of inferencing. |
|
Enterprise and systematic approach to closing the loop between AI and human. |
Communicate with AI enterprise wide. Human and AI as interacting partners through feedback integration APIsb for enterprise-wide reusability. |
Note a: Use case five is not AI. Rather, it involves the development of a model monitoring system.
Note b: An application programming interface (API) is a software intermediary that allows applications to communicate with each other.
Source: ATO’s A&AI strategy.
Implementation arrangements
2.24 On several occasions throughout the development of the A&AI strategy, the ATO committee overseeing the development of the strategy sought clarity on implementation. The A&AI strategy did not set out implementation arrangements. It stated that the next steps would involve the development of implementation arrangements including: developing a plan; scoping and designing value cases for each enterprise use case; implementing use cases; reporting on value returned; and proceeding with other future use cases.
2.25 Between October 2022 and July 2024, the ATO acknowledged the need to develop overarching implementation arrangements for the A&AI strategy. On 23 July 2024, the ATO provided the ANAO with a document titled ‘A&AI Implementation plan’ dated January 2024. The ATO described this document as ‘a high-level implementation plan designed for an executive audience’ and noted that further detailed planning is needed. Approval of this document was not evident.
2.26 Implementation of the A&AI strategy is the responsibility of the ATO’s Smarter Data area. The Assistant Commissioner Data Science within Smarter Data is nominated as the senior responsible officer for implementation of the strategy. Roles and responsibilities supporting the implementation of the strategy across the ATO have not been clearly defined.
2.27 According to the ATO’s policy on corporate project management, a corporate project is a body of work that requires resourcing or services that cannot be funded or sourced from within a single business line. While this is the case for the A&AI strategy which has funding sources from multiple business areas, implementation of the A&AI strategy is not being managed as a corporate project. The ATO is implementing the A&AI strategy as a business line project.53 The ATO’s guidance for business line projects require a project outline be completed to document how the project will be managed. A project outline had not been developed for the A&AI strategy.
2.28 On 22 May 2024, the Strategy Committee recommended that delivery of the five use cases should continue with current funding and resourcing (this includes a mix of business-as-usual funding and funding from new policy proposals).
Recommendation no.1
2.29 The ATO align implementation arrangements for the automation and AI strategy with enterprise-wide requirements.
Australian Taxation Office response: Agreed.
2.30 The ATO will undertake work to improve the alignment of the automation and AI strategy with enterprise requirements, noting we may need to subsequently evolve it to align with the rapidly changing AI environment and any APS requirements as they are developed.
Has the ATO clearly defined and communicated roles and responsibilities supporting the adoption of artificial intelligence?
The ATO uses existing organisational and governance structures to support its adoption of AI, and it has been adapting these for AI. Over time, the ATO has also established AI-focussed governance bodies. Key roles and responsibilities with respect to AI are not always clearly defined, including enterprise-wide responsibilities and accountabilities over the ATO’s AI governance framework and for AI models and systems. In September 2024, the ATO established a Data and Analytics Governance Committee in recognition that stronger governance arrangements were needed. In November 2024, the ATO appointed its Chief Data Officer as its accountable official under the Policy for the responsible use of AI in government.
2.31 A part of good governance is ensuring that responsibilities and accountabilities, including decision-making and oversight roles, are clearly defined and communicated.54 For AI, ‘existing decision-making and accountability structures should be adapted and updated to govern the use of AI’.55 It should be clear who is responsible and accountable for determining and implementing an entity’s overarching approach to AI and for individual AI systems or uses of AI.56
Organisational structures
2.32 The ATO advised the ANAO on 19 April 2024 that key AI responsibilities are within the Client Engagement Group, and the Enterprise Solutions and Technology Group.
2.33 Within the Client Engagement Group, the Smarter Data area57 has primary responsibility for the ATO’s management and governance of data and analytics, including AI activities. The Data Science branch in Smarter Data provides skills and advice on AI, machine learning, deep learning and natural language processing. In January 2024, the ATO established an AI Governance team within the Data Management branch in Smarter Data. The Deputy Commissioner Smarter Data is responsible for:
- guiding strategic data management policies, practices and procedures;
- ensuring consistent data governance and management across the ATO; and
- the management of two AI-related enterprise risks: the ‘maximising the value of data and analytics’ risk and the ‘misuse of data and analytics’ risk (see Table 2.4).
2.34 Within the Enterprise Solutions and Technology Group, key responsibilities relating to AI relate to technology solutions and ICT architecture, cyber security governance and operations.
Enterprise-level governance committees
2.35 The Commissioner of Taxation (the Commissioner) is the accountable authority of the ATO and is supported by the ATO Executive Committee. The ATO’s Audit and Risk Committee supports the Commissioner by providing independent advice and assurance.58 The Commissioner and the ATO Executive Committee are supported by six enterprise-level committees: Finance Committee; People Committee; Risk Committee; Security Committee; Strategy Committee and National Consultative Forum.59
2.36 The ATO’s Risk Committee and Strategy Committee have roles in relation to AI.
- The Risk Committee is responsible for oversight of the management of enterprise risks including the ‘misuse of data and analytics’ and the ‘maximising the value of data’ risks.
- The Strategy Committee is responsible for overseeing the implementation of the ATO’s A&AI strategy. It also has responsibilities in relation to data and analytics including ensuring that ATO strategies and programs are data and analytics driven, and overseeing data ethics and data stewardship issues.
2.37 The ANAO reviewed meeting minutes of the ATO Executive Committee, the Audit and Risk Committee, the Risk Committee and the Strategy Committee between 1 July 2022 and 30 June 2024 to understand how these committees have considered the ATO’s adoption of AI.60 A summary of this analysis is presented in Table 2.3. AI was discussed at and reported to these committees on a range of occasions, although reporting was not based on defined and agreed arrangements.
Table 2.3: ATO governance committees’ oversight of the ATO’s adoption of AI
Committee and summary of discussion related to AI, July 2022 to June 2024 |
The Commissioner and the ATO Executive Committee — the ATO Executive Committee discussed AI five times. Discussions included: AI in the context of a scan of business community concerns; review of governance arrangements to manage proposals and the application of AI; two briefings in relation to generative AI; and the ATO’s A&AI strategy. These discussion items were initiated by the Audit and Risk Committee, the Risk Committee and the Chief Financial Officer. |
Audit and Risk Committee — Discussions included: the use of AI in a service delivery context; the ATO’s two AI-related enterprise risks, the inclusion of AI in the ATO’s data management policy; the ATO’s policy on the use of publicly available generative AI; the impact of AI on emerging data and technology-related risks; whole-of-government AI initiatives; and audits of AI as part of the internal audit program. |
Risk Committee — the Risk Committee discussed AI on five occasions. Discussions included: the risks and opportunities for the use of generative AI across the ATO; the two AI-related enterprise risks; and a proposal to establish a data and analytics governance committee. |
Strategy Committee — the Strategy Committee discussed the A&AI strategy on three occasions. It also discussed the opportunities and risks related to generative AI technologies and, more broadly, the potential use of AI by those in the taxation and superannuation systems for ‘nefarious purposes’. |
Source: ANAO analysis of ATO documents.
AI roles and responsibilities
AI governance committees
2.38 Over time, the ATO has established governance bodies with areas of focus relating to AI. In September 2024, the ATO established the Data and Analytics Governance Committee. The committee’s role is to make decisions and provide to promote the responsible use of data and analytics at the ATO. The charter notes that this role will evolve over time. It reports to the Strategy Committee.
2.39 In establishing this committee, it was noted that ‘continued fragmentation of approaches to [data and analytics] governance and management’ was a risk of not establishing the committee. From October 2024, the following extant groups report to the Data and Analytics Governance Committee.
- The Business Automation Governance Committee (established in June 2021) is to govern and oversee business automation decisions and to ‘fulfil the A&AI strategy’. The Committee’s role in relation to the A&AI strategy is not clearly defined. The ATO advised the ANAO on 8 August 2024 that it has no formal role in relation to the strategy.
- The Data Ethics Review Panel (established in November 2021) is as an escalation point for data ethics issues. The panel is convened as needed. As of April 2024, it had not been convened.
- The Generative AI Senior Executive Service Band 2 Group (Gen AI B2 Group) was established in November 2023 to oversee the ATO’s use of generative AI technology. It is responsible for the ATO’s ‘Use of publicly available generative AI technology policy’. The Gen AI B2 Group61 reports to the Risk Committee.
Accountable officials and other expertise
2.40 The Policy for the responsible use of AI in government requires that entities designate accountability for implementation of the policy to accountable officials by 30 November 2024.62 The responsibilities of accountable officials ‘may be vested in an individual or in the chair of a body. The responsibilities may also be split across officials or existing roles (such as Chief Information Officer, Chief Technology Officer or Chief Data Officer) to suit agency preferences’. In November 2024, the ATO appointed its Chief Data Officer (Deputy Commissioner Smarter Data) as its accountable official.
2.41 The National framework for the assurance of artificial intelligence in government outlines that AI ‘requires a combination of technical, social and legal capabilities and expertise … such as data and technology governance, privacy, human rights, diversity and inclusion, ethics, cyber security, audit, intellectual property, risk management, digital investment and procurement’.63
Responsibilities and accountabilities — system level
2.42 The accountability principle of Australia’s AI ethics principles states that ‘people responsible for the different phases of the AI system lifecycle should be identifiable and accountable for the outcomes of the AI systems, and human oversight of AI systems should be enabled’.64
2.43 The ATO’s data stewardship model is about defining the accountabilities and responsibilities around the management and oversight of the ATO’s data assets. The ATO has identified that further work is needed to fully implement stewardship for AI outputs.
Recommendation no.2
2.44 The ATO clearly define and communicate enterprise-wide organisational structures and governance arrangements supporting its adoption of AI, including defining accountabilities and responsibilities at the model and system level.
Australian Taxation Office response: Agreed.
2.45 The ATO will define and communicate enterprise-wide organisational structures and governance arrangements supporting its adoption of AI, including defining accountabilities and responsibilities at the model and system level.
Does the ATO have effective arrangements for managing risks in relation to the adoption of artificial intelligence?
There are risks related to the adoption of AI at various levels at the ATO.
- The ATO has two enterprise risks that relate to AI due to their focus on data and analytics. These risks are ‘above tolerance’.
- The ATO has risk assessment processes that apply to its adoption of AI. It has identified that these are not sufficient for AI-specific risks, and it is working to introduce processes that better support the management of AI risks.
- The ATO has a risk-based approach to approving the use of publicly available generative AI technologies by ATO officers.
2.46 An accountable authority must establish and maintain appropriate systems of risk oversight and management and internal control for their entity.65 The ATO is required to comply with the Commonwealth Risk Management Policy. The National framework for the assurance of artificial intelligence in government outlines that ‘risks should be managed throughout the AI system life cycle’ and that ‘monitoring and feedback loops should be established to address emerging risks, unintended consequences or performance issues’.66
Enterprise risk management — AI-related enterprise risks
2.47 The ATO’s Risk Management Chief Executive Instruction67 and Enterprise Risk Management Framework (ERMF) set out the ATO’s enterprise-wide approach to risk management. The ATO has a register of its enterprise risks.68
2.48 The ATO advised the ANAO on 12 March 2024 that two of its enterprise risks are related to AI due to their focus on data and analytics (see Table 2.4).69 This section focusses on these two risks.
Table 2.4: The ATO’s AI-related enterprise risks
Description of risk event |
Control effectivenessa |
Risk levelb |
Tolerancec |
Maximising the value of data and analytics |
|
|
|
There is a risk that the ATO does not effectively utilise data and analytics capabilities, caused by inappropriate investment in or maintenance of data and analytics foundations and capabilities, resulting in sub-optimal decision-making, organisational inefficiency and uneconomic outcomes. |
Partially effective |
High |
Above tolerance as target risk leveld is ‘medium’ |
Misuse of data and analytics |
|
|
|
There is a risk that the ATO (or those the ATO shares data or analysis with) does not lawfully or appropriately use its data and analysis, caused by a failure in its or its partners’ data and analytics governance, resulting in adverse impacts on individuals, loss of revenue and/or loss of public trust and confidence and reduction in willing participation. |
Partially effective |
Medium |
Above tolerance as target risk leveld is ‘low’ |
Note a: Controls are assessed as: effective; partially effective; ineffective; and insufficient evidence.
Note b: The risk level is based on the consequence if the risk is realised and the likelihood that the risk will be realised. The ATO’s risk levels categories are: very low; low; medium; high; very high and extreme. The ATO updated its risk level and consequence category names in August 2024.
Note c: The tolerance rating is either within tolerance or above tolerance. Above tolerance means that the risk rating is higher than what is acceptable.
Note d: Target risk refers to the level of risk willing to be tolerated for a risk.
Source: ATO documentation.
2.49 The ATO’s risk appetite statement states that it is willing to accept higher levels of risk where there is a clear opportunity to realise benefits and where risks can be controlled to acceptable levels. It is less willing to accept risk where it is not clear that benefits will be realised or where risks are unable to be controlled to acceptable levels. The risk appetite statement provides a sound basis to guide its approach to managing the AI-related enterprise risks. The ATO has identified that failing to appropriately treat the ‘maximising the value of data and analytics’ risk could result in ‘sub-optimal outcomes or opportunities lost’ and failing to appropriately treat the ‘misuse of data and analytics’ risk could result ‘in actual harm’.
Responsibilities
2.50 Roles for managing the AI-related enterprise risks are defined in accordance with requirements. Under the ERMF, risk owners of enterprise risks are ‘personally accountable’ for risk management, provide direction on relevant risk management activities and oversee the status of risks, controls and treatment strategies. The Deputy Commissioner Smarter Data is the risk owner for the two AI-related enterprise risks. In accordance with the ATO’s ERMF, each of these risks also has a risk manager, and control and treatment owners.
Risk assessment
2.51 The ‘misuse of data and analytics’ risk was categorised as an enterprise risk in March 2023. The ‘maximising value of data and analytics’ risk was updated in May 2023 to include ‘analytics’ as part of its scope. The following paragraphs examine the management of these risks.
Maximising the value of data and analytics risk
2.52 There were nine controls allocated to the ‘maximising the value of data and analytics’ risk. Overall, these controls were rated as ‘partially effective’. One of the ATO’s controls had a direct link to the ATO’s adoption of AI and was about the implementation of the five A&AI strategy use cases. This control was identified as supporting the ATO to leverage investment across the organisation by providing access to A&AI solutions more efficiently and quickly.
2.53 The ATO had not assessed the effectiveness of this control due to ‘insufficient evidence’.70 The ATO’s assessment indicated a lack of progress in implementing the use cases of the A&AI strategy.
2.54 Overall, the risk was rated as high. The tolerance for this risk (target risk level) was ‘medium’ which means that this risk was above tolerance. As controls were ‘partially effective’ and the risk was tracking above tolerance, the ATO has allocated a risk treatment which focusses on the ATO’s Data and Analytics Strategy, including delivering the A&AI strategy. The treatment owner for this risk was the Deputy Commissioner Smarter Data. The due date for this treatment was listed as 31 December 2024 in the enterprise risk register and 31 December 2027 in the risk assessment and treatment plan. The ANAO sought clarification from the ATO about the due date for this treatment. The ATO advised the ANAO on 16 September 2024 that it anticipates that both enterprise risks will be within tolerance by the completion of the next Data and Analytics Strategy which is planned for 2029.
Misuse of data and analytics risk
2.55 There were 17 controls allocated to the ‘misuse of data and analytics’ risk. These controls were rated as ‘partially effective’ overall. One control was directly relevant to the ATO’s adoption of AI — the implementation of a model ethics assessment process for models containing automation, AI or machine learning. It was described as aiming to detect the possible misuse of data and analytics, including AI, in the design and implementation of models. This control was assessed as having ‘insufficient evidence’ to be able to be assessed as the model ethics assessments were in pilot phase at the time of assessment. See paragraph 2.81 for further discussion on the model ethics assessment process.
2.56 Overall, the ‘misuse of data and analytics’ risk was rated as medium. The tolerance for this risk was ‘low’ which means that this risk was above tolerance. As controls were ‘partially effective’ and the risk was tracking above tolerance, the ATO has allocated a treatment for the risk — the same treatment as for the ‘maximising the value of data and analytics’ risk which relates to the implementation of the ATO’s Data and Analytics Strategy and the A&AI strategy.
Shared risks
2.57 The ATO has categorised the two AI-related enterprise risks as shared71 with other Australian Government entities that receive or use its data or analytical outputs. According to the ATO’s guidance, shared risks require shared oversight and management between entities.72 Arrangements for managing these shared risks have not been established.
Monitoring and review
2.58 The ATO’s risk management guidance states that risks should be monitored on an ongoing basis and periodically reviewed. Monitoring and review should be planned, with clearly defined responsibilities. The ‘maximising the value of data and analytics’ risk should have been reviewed in August 2024 and the ‘misuse of data and analytics’ risk in February 2024. Both risks were reviewed in December 2024.
2.59 Commonwealth entities are required to establish processes for identifying, managing and escalating emerging risks.73 The ATO has identified that of its data and analytics activities74, AI activities have the highest overall risk, with a particularly high risk for the ‘misuse of data and analytics’ risk. This is due to the combination of autonomy and adaptivity and lack of controls. This highlights the importance of ongoing monitoring and review of the two AI-related enterprise risks as they are likely to continue to evolve.
2.60 ATO risk management guidance outlines that reporting on risks is ‘an integral part of governance’.
- There was no structured and regular reporting on the two AI-related enterprise risks to the risk owner. The ATO advised the ANAO in January 2025 that since June 2024 the risk manager for the two AI-related risks ‘generally meets with the risk owner on a fortnightly basis’.
- In November 2023 and September 2024, the risk owner for the two AI-related risks provided an update to the Risk Committee, including an overview of the risks, and work underway to mature controls and treatment strategies. The September 2024 reporting provided more information about the environmental factors and drivers of the risks, the controls in place and the treatments needed to bring the risks in tolerance.
- The ATO’s Audit and Risk Committee receives reporting on all enterprise risks, including the two AI-related enterprise risks. This reporting has improved over time.
Recommendation no.3
2.61 The ATO review the ‘misuse of data and analytics enterprise’ risk in accordance with its enterprise risk management framework and risk appetite, and update and incorporate controls relating to the impact of AI on this risk.
Australian Taxation Office response: Agreed.
2.62 The ATO will review the misuse of data and analytics enterprise data risk in accordance with the ATO Risk Management Framework and update and incorporate controls relating to the impact of AI.
Arrangements for managing AI business risks
2.63 Under the Commonwealth Risk Management Policy, ‘risk management must be embedded into the decision-making activities of an entity’.75 The National framework for the assurance of artificial intelligence in government outlines that the use of AI should be risk based.76 According to the OECD:
[o]rganisations should devise, adopt and disseminate a combination of risk management policies that articulate an organisation’s commitments to trustworthy AI. These policies should be embedded into an organisation’s oversight bodies.77
2.64 At the ATO, business risks are all other risks (i.e. not enterprise) associated with day-to-day operations. Business risks may be at the group, business line, team or project level. There is no central register of business risks, although these risks can be added to the enterprise register. The ATO advised the ANAO on 12 April 2024 that there are no AI-related business level risks in the enterprise risk register. The ATO also advised that risks may be managed at the project level. It did not identify any such risks.
Risk management throughout the AI system lifecycle
2.65 The ATO’s organisational risk appetite statement notes that it is willing to accept higher risk if there are clear opportunities and where risk can be controlled to an acceptable level. Except for the process to assess publicly available generative AI tools for use by ATO officers (see paragraphs 2.69 to 2.73), as of July 2024, the ATO did not have a defined process for assessing the risk level of AI models and systems.78
2.66 The ATO has identified that its current arrangements ‘do not sufficiently capture AI specific risks’. As at July 2024, the ATO was developing AI risk management guidance which is planned to include an AI risk assessment approach. The guidance will aim to assist ATO officers assess whether proposed AI systems are within risk tolerance and whether governance is being applied proportionate to the risk. The ATO’s desired future state includes that governance and processes for AI will be tailored and based on assessed risk.
2.67 As at July 2024, the ATO had a variety of processes that capture or assess risks related to AI. This includes: data and analytics ethics assessments79; privacy impact assessments; security risks assessments; and risk assessments as part of project management and model design documentation.
2.68 In chapters 3 and 4, the ANAO examines the ATO’s processes for designing, developing, deploying and monitoring AI models. Risks should be assessed and managed throughout the AI system lifecycle.80 As of July 2024, the ATO was also considering how it can integrate risk management into existing processes.
Use of publicly available generative AI
2.69 The ATO has identified the use of generative AI by its officers as a business risk. In September 2023, the ATO conducted a cyber security risk assessment of two generative AI platforms. This risk assessment focussed on the security implications of using publicly available generative AI, and noted that neither of the platforms ‘are recommended as secure for use within the ATO or with ATO data (including the registration of accounts with ATO email addresses)’.
2.70 The ATO commenced drafting a risk assessment and treatment plan for this risk in September 2023. It assessed that the elements of this risk related to security, potential for biased or inaccurate outputs from platforms, impact on accurate decisions and lack of transparency and explainability of outputs. As at July 2024, the assessment had not been finalised.
2.71 As discussed at paragraph 2.9, the ATO has a risk-based policy and process for approving the use of publicly available generative AI by its officers. Under the policy, ATO officers may apply to have generative AI tools approved for work purposes where the risk of negative impact is low.
- Officers must assess the benefits and risks of their proposals. The assessment of risk is around: privacy; technology; data; transparency; and cost.
- Applications are to be sponsored by an SES Band 1 officer, reviewed by the Generative AI Band 1 Working Group and then either approved or rejected by the Gen AI Band 2 Group.
2.72 As of 18 June 2024, there were eight publicly available generative AI tools approved for use in the ATO (see Table 2.1).
2.73 While the ATO did not finalise its risk assessment and treatment plan for the generative AI-related business risk, this risk warrants ongoing monitoring and review. The charters for the Generative AI Band 1 Working Group and the Generative AI Band 2 Group specify responsibilities for these groups to monitor the risks related to the ATO’s use of generative AI.
Opportunity for improvement
2.74 The ATO could finalise the risk assessment and treatment plan for the use of generative AI business risk and establish ongoing monitoring and review arrangements for this risk and the ATO’s use of publicly available generative AI policy.
Does the ATO have effective arrangements to support the implementation of artificial intelligence that aligns with ethical principles?
The ATO’s data ethics framework aims to support the ATO to deliver ethical data activities, including AI. For its AI models, the ATO has not complied with the requirements of this framework (74 per cent of AI models in production did not have completed data ethics assessments). This undermines the ATO’s ability to deliver and to assure the delivery of AI that aligns with ethical principles. The ATO has not developed effective monitoring and assurance arrangements for its data ethics framework.
2.75 Developing and using AI in an ethical manner is an important part of building public trust in the use of AI by public sector entities. Australia’s AI Ethics Principles (see Figure 1.1) provide a framework to support the ethical design, development, deployment and use of AI.
The ATO’s data ethics framework
2.76 The ATO has developed a data ethics framework to support the delivery of data activities, including AI, that meet ethical principles. Since January 2022, the framework has been mandatory for all ATO data activities81, including AI developed in house or procured by the ATO. The data ethics framework outlines the ‘systems of practices and procedures that help guide our actions’ and ‘demonstrat[e] adherence to our data ethics principles’ and includes: principles; ethics threshold and impact assessments; guidance; and governance.
Principles
2.77 The data ethics principles (see Box 2) were endorsed by the ATO Executive Committee in November 2020 after internal and public consultation. The ATO’s six data ethics principles provide guidance on the behaviours expected when conducting data and related activities.
Box 2: The ATO’s data ethics principles |
|
Source: ATO, How we use data and analytics, 2022, available from https://www.ato.gov.au/about-ato/commitments-and-reporting/information-and-privacy/how-we-use-data-and-analytics [accessed 23 May 2024].
2.78 Aspects of Australia’s AI Ethics Principles which are not addressed by the ATO’s data ethics principles include reproducibility (part of the reliability and safety principle82) and auditability (part of the accountability principle83) of AI system outputs.
2.79 Australia’s AI Ethics Principles do not have a specific principle related to legality.84 Principle 2 of the ATO’s data ethics principles is ‘uphold privacy, security and legality’. This principle states that the ATO ‘ensures that the individual and community information we hold is kept safe, protected and shared securely as authorised by law.’ ATO officers are to assess whether AI activities are ‘permissible by law’.
Ethics threshold assessment and impact assessment
2.80 As part of the data ethics framework, ATO officers are required to assess ethical considerations for data activities. Since January 2022, all new projects and any new or significantly changed data activities must complete a data ethics assessment. There are four components to the ATO’s ethical assessments:
- the data ethics threshold assessment (DETA) and the data ethics impact assessment (DEIA) — see Box 3; and
- the model ethics threshold assessment (META) and the model ethics impact assessment (MEIA) — see Box 4.
Box 3: Data ethics assessments |
|
2.81 In August 2021, the ATO identified that the DETA and DEIA were not sufficient for analytical models, including AI, due to the lack of processes and guidance to support the assessment of ethical considerations for AI models. In April 2022, the ATO commenced piloting a supplementary process to assess ethical considerations for modelling, AI and algorithmic activities (models ethics assessments). The pilot finished in August 2023. The ATO released a revised data ethics assessment on 26 August 2024 (the data and analytics ethics assessment), which includes the four components of the ethical assessments (DETA, META, DEIA and MEIA) in a single assessment template.
Box 4: Model ethics assessments |
|
Guidance
2.82 The ATO’s Data Ethics Behavioural Guidelines aim to assist ATO officers apply the data ethics framework, including by providing examples of relevant behaviours and guidance on how to address the data ethics questions.
2.83 In August 2024, with the release of the data and analytics ethics assessment (see paragraph 2.81), the ATO released a revised guide (the Data and Analytics Ethics Assessment User Guide) to support officers assess ethics considerations for its data and models. The user guide notes that it is a ‘living document’ that will be updated as advice changes or new content needs to be included.
Governance
2.84 Key roles and responsibilities set out in the data ethics framework include the following.
- The data ethics framework is owned by the Deputy Commissioner Smarter Data.
- Senior Accountable Officers, also known as data leaders, own ethics risks and are responsible for risk mitigation strategies and making decisions about these risks.
- The data ethics team in Smarter Data85 has an advisory and escalation role. ATO officers can request advice on ethics assessments and related risk management.
- The Data Ethics Review Panel is to be convened if data ethics issues cannot be resolved or warrant further consideration or escalation.
2.85 The ATO advised the ANAO in July 2024 that several learning and development products support implementation of the data ethics framework. These products include: ‘Working in the ATO’; ‘Safe, Secure and Inclusive’; and ‘Data Ethics Intermediate Training’.86
2.86 Similar to ‘privacy by design’87 and ‘secure by design’88 processes, ethical considerations should be embedded into the design of AI models and systems. The ATO aims to ensure that data ethics is ‘built into corporate processes’.
- In February 2024, the ATO included references to the data ethics framework in corporate project management processes.
- For business line projects, ATO guidance suggests that the ethics assessments should be completed and approved prior to deployment of an AI model.
Completion of ethics assessments — AI models
2.87 As outlined in Table 2.1, as of 14 May 2024, the ATO had 43 AI models in production. For these 43 AI models, as of August 2024, the ATO had finalised:
- two data ethics assessments (covering 1189, or 26 per cent of, AI models) — 32 (74 per cent) models did not have an assessment; and
- two model ethics assessments (covering two AI models — five per cent).90
2.88 The ANAO requested that the ATO provide an explanation as to why data ethics assessments have not been completed for AI models in production.
- As stated in paragraph 2.80, data ethics assessments have been required for ‘new’ and ‘significantly changed’ data activities and projects since January 2022.
- Of the 43 models in production, 22 were introduced after January 2022 and were ‘new’. Of these 22, 10 (45 per cent) had data ethics assessments.
- Of the other 21 models in production, the ATO advised the ANAO on 21 October 2024 that it considered that two of these models had ‘significantly changed’ since January 2022. Neither of these models had a data ethics assessment. The ATO’s assessment that two of the 21 models have significantly changed is not consistent with ATO guidance which states that ‘significantly changed’ includes ‘changes or updates to the data, model or model suite’. On this aspect, the ATO advised that not all updates to data will result in a significant change. Rather, changes that have the potential to impact identified data ethics risks, or to introduce new risks are considered significant.
Monitoring and assurance arrangements
2.89 Monitoring and assurance arrangements should be designed to assess whether AI is being delivered ethically.91 Under the ATO’s data ethics framework, there are to be arrangements for the ‘assurance and confidence in the application [of the framework]’.
2.90 As of July 2024, the ATO has not established monitoring and assurance arrangements over its data ethics framework for its AI models, including over the completion and approval of assessments. The data ethics team maintains a register of data ethics assessments that have commenced or have been completed. It does not monitor whether assessments have been completed in accordance with requirements.
2.91 The National framework for the assurance of artificial intelligence in government advises that governments should ‘test and verify the performance of AI systems’ and that the ‘use of AI is continuously monitored and evaluated to ensure its operation is safe, reliable and aligned to ethics principles’.92
Recommendation no.4
2.92 The ATO improve its arrangements in support of the design, development, deployment and use of AI that aligns with ethical principles by:
- specifying requirements relating to AI reproducibility and auditability;
- ensuring the data ethics framework is integrated into other ATO processes;
- completing ethics assessments for the AI models in production; and
- introducing monitoring, assurance and reporting arrangements over the implementation of its data ethics framework.
Australian Taxation Office response: Agreed.
2.93 The ATO will improve its arrangements in support of the design, development, deployment and use of AI that aligns with ethical principles.
3. Arrangements supporting the design, development and deployment of artificial intelligence models
Areas examined
This chapter examines whether the Australian Taxation Office (ATO) has established arrangements to support the design, development and deployment of artificial intelligence (AI) models.
Conclusion
The ATO has partly effective arrangements supporting the design, development and deployment of its AI models.
- The ATO does not have specific policies and procedures for the design, development and deployment of its AI models, although there are enterprise policies and procedures which are relevant. The lack of approved and embedded policies and procedures creates risks to the effective implementation of models.
- The ATO has not sufficiently integrated ethical and legal considerations into its design and development of AI models. This impairs the ability of the ATO to demonstrate that its AI models are: fair and free from bias; reliable and safe; protecting privacy; transparent and explainable; contestable; and have appropriate accountability arrangements.
- There are no clearly defined assurance and approval arrangements that set out testing, validation, review and decision-making throughout the design, development and deployment of AI models.
Area for improvement
The ANAO made one recommendation aimed at establishing and implementing policies and procedures for the design, development and deployment of AI models.
3.1 The concept of an AI system lifecycle refers to the phases involved in turning a business problem into an AI solution. Appendix 6 depicts two AI system lifecycles. The Organisation for Economic Co-operation and Development states that organisations implementing AI systems must:
take measures [to] ensure their AI systems are trustworthy — i.e. that they benefit people; respect human rights and fairness; are transparent and explainable; and are robust, secure and safe. To achieve this, actors need to govern and manage risks throughout their AI systems’ lifecycle — from planning and design, to data collection and processing, to model building and validation, to deployment, operation and monitoring.93
Has the ATO established effective arrangements for the design of artificial intelligence models?
The ATO has not established a framework of policies and procedures for the design of AI models. For the 14 models that the ATO developed and deployed between 1 July 2023 and 14 May 2024, there were mixed practices related to planning, governance and design. The ATO largely defined business problems to be solved by AI models, defined roles and responsibilities and documented stakeholder engagement. There were gaps in terms of: project planning and risk management; assessment of ethical, security, privacy and legal considerations; and assurance, decision-making and record keeping. For the work-related expenses AI models, there were gaps in how the ATO assessed ethical, privacy and legal considerations.
Policies and procedures
3.2 The ATO has not developed a framework, including policies and processes, for designing its AI models. The ATO advised the ANAO on 10 May 2024 of the steps it takes to design models (see Box 5). Some of these steps are based on the ATO’s IT Delivery Method.94
Box 5: Advice from the ATO about its approach to designing AI models |
|
Note a: ‘Business’ refers to the ATO officers who will be using the outputs of the AI model.
Note b: This is different to a data ethics impact assessment outlined in Box 3.
Source: ATO advice and documentation.
3.3 In addition to the advice in Box 5, AI models may be developed as part of ATO project management methodologies. The ATO requires that its IT Delivery Method is used for projects which have an IT component.
3.4 The steps described in Box 5 provide aspects of a design process. The ANAO compared the ATO’s advice with a range of relevant standards, frameworks, guidelines and policies and observed the following.
- The ATO has not defined the minimum documentation requirements of the design phase, including both technical and non-technical design documentation, and record keeping arrangements for design documentation.95
- The ATO has not defined how the ethics principles are to be considered throughout the design phase and other phases of the AI system lifecycle.96
- Risk management could be better embedded throughout the design phase.97
- Assurance and decision-making responsibilities are not clearly defined.98
Privacy and security — platform for model development
3.5 The ATO’s Advanced Analytics Platform (AAP) is a platform for the ATO’s data scientists to develop, train and execute data and analytics models including machine learning, deep learning, neural networks, forecasting and pattern matching. Since December 2022, the AAP has operated in a cloud environment.99 The AAP environment is separated from other on-premises and cloud environments used by the ATO, however, it can connect to selected sources of data which host sensitive information.
3.6 Given the role of the AAP, the Protective Security Policy Framework (PSPF) requires that the Commissioner of Taxation, the Chief Information Security Officer or their delegate provide an authorisation to operate prior to use.100 The ATO refers to this authorisation as a Security Authorisation to Operate (SATO). The ATO has also assessed that obligations under the Privacy Act 1998 to protect personal information collected by the ATO are relevant to the use of the AAP.
3.7 The SATO for the AAP was approved on the basis that activities would be completed to confirm the effectiveness of security controls relied upon by the SATO. These activities were also relevant to confirm the effectiveness of controls addressing the ATO’s Privacy Act 1988 obligations.101 The ATO did not complete all of these activities prior to bringing the AAP into use, and as at June 2024, they had not been completed or planned.
3.8 The PSPF also requires that entities manage security risks for ICT systems on an ongoing basis.102 The ATO has not consistently monitored the effectiveness of security controls while the AAP has been in operation. The ATO receives regular reports from its cloud provider attesting to the sufficiency of the providers security controls during the reporting period and any identified exceptions to these. These reports were not reviewed by the ATO for a period of two years.103
3.9 The ATO developed a system security plan describing how the AAP addresses relevant security controls of a 2019 version of the Information Security Manual. It has not reviewed the system security plan since then to confirm if controls remain appropriate.104 Regular review of security plans and service provider reports is important to ensure that controls remain effective and appropriate as circumstances change.
Designing models in practice
3.10 The ANAO examined the design practices for the 14 AI models deployed between 1 July 2023 and 14 May 2024. Overall, the ANAO found mixed practices with regards to the planning, governance and design of AI models. Table 3.1 provides a summary of the ANAO’s assessment (as of June 2024).
Table 3.1: Assessment of the planning, governance and design of the ATO’s AI models
Componenta |
ANAO assessment |
Business problem |
For 12 (86%) of the 14 AI models, business problems were clearly defined in PL191s, although 15 (45%) of the 33 PL191sb were not finalised (see Table 3.2). Two models did not have PL191s. |
Roles and responsibilities |
Relevant business areas were not always allocated key roles and responsibilities. For four (29%) of the 14 AI models, roles were not clearly defined. It was not evident that there was sufficient consideration given to specifying experts in relation to ethics, privacy, law conformance and other relevant areas. |
Planning |
For the 14 AI models, there were five projects. Of the five projects, four (80%) had project plans and one (20%) did not. One (7%) AI model had up to date planning documentation and evidence of approval and 13 (93%) did not. |
Risk management |
Twelve (86%) of the AI models included consideration of risks and two (14%) did not. The ATO advised the ANAO on 16 September 2024 that there were no risks related to the development of these two models. The ATO did not provide evidence of how this assessment was made. |
Ethical, privacy, legal and security |
|
Stakeholder engagement |
The ATO provided evidence of regular internal stakeholder engagement for 11 AI models and no evidence of internal stakeholder engagement for three AI models. |
Note a: The components in this table were informed by: the AS ISO/IEC 42001:2023 Information technology — Artificial intelligence — Management system standard; Australia’s AI Ethics Framework; and the National framework for the assurance of artificial intelligence in government.
Note b: Models may have more than one PL191.
Note c: To meet the Privacy Act 1988, a privacy impact assessment (PIA) is to be conducted for all high privacy risk activities (where determined through a PIA threshold assessment).
Note d: Three privacy impact threshold assessments were completed to assess 10 models in the same project.
Source: ANAO analysis of ATO documentation.
3.11 Table 3.2 provides a summary of the ANAO’s assessment of the assurance, decision-making and record keeping of the design phase for the 14 AI models deployed between 1 July 2023 and 14 May 2024. The ANAO found that there are areas requiring improvement.
Table 3.2: Assurance, approval and record keeping of the ATO’s design of AI models
Componenta |
ANAO assessment |
Assurance |
For the design phase, the ATO did not define assurance arrangements. Evidence of assurance provided by the ATO included review of the PL191s by relevant officers and emails with review, analysis and testing covering 11 (79%) of the 14 models. |
Decision-making |
Approval of the design phase is to be documented in the PL191. Across the 14 AI models, the ATO provided 33 PL191s.b
The ATO advised the ANAO on 14 October 2024 that PL191s are finalised on delivery of a model and updates and finalisation may be recorded elsewhere. The ATO has acknowledged the need for improvements in information management. |
Record keeping |
Record keeping for the design phase was not adequate. As noted above, 45% of the PL191s for the 14 AI models were in draft. Project plans were in draft for 11 (79%) AI models and three AI models did not have appropriate planning documentation. |
Note a: The components in this table were informed by: the AS ISO/IEC 42001:2023 Information technology — Artificial intelligence — Management system standard; Australia’s AI Ethics Framework; and the National framework for the assurance of artificial intelligence in government.
Note b: Models may have more than one PL191.
Source: ANAO analysis of ATO documentation.
Work-related expenses artificial intelligence models
3.12 Work-related expenses (WRE) are expenses incurred by taxpayers, and not reimbursed, to earn their income or when performing their work.105 WRE claims are the single largest contributor to the tax gap106 for individuals not in business.107
3.13 In July 2020, the ATO commenced the Streamlining Substantiation of Deductions project to address the tax gap related to WRE claims by individuals not in business. The project includes the development of AI models to predict and support the treatment of risks associated with WRE claims. Throughout the audit report, these are referred to as the WRE AI models. Appendix 4 provides an overview of the four WRE AI models.
3.14 The ANAO examined aspects of the design of the WRE AI models, with a focus on how the ATO defined the business problem and selected model attributes, and how it has assessed ethical considerations, including privacy and legality.
Defining the business problem and selection of AI model attributes
3.15 AI models should be designed in collaboration with the project owner, or business area, to establish required technical and functional attributes for model design.108 Case study 1 outlines the business problem and selection of model attributes for the WRE AI models.
Case study 1. Defining the business problem and selecting attributes for the WRE AI models |
The ATO has identified that there is significant non-compliance in relation to WRE claims. In 2021–22, the ATO estimated that individuals not in business accounted for $10.2 billion of the net tax gap and $3.6 billion (35 per cent) was related to incorrect claims of WRE.a As of September 2022, the ATO was limiting the number of audits of WRE claims per year due to the availability of human resources to conduct manual reviews and audits. The ATO conducts other pre-lodgment compliance activities through education (such as communication products and online tools) and targeted nudge messages (such as prompt messages to support taxpayers to comply). The ATO commenced developing the WRE AI models in 2020–21 to address the tax gap resulting from non-compliant WRE claims. Expected benefits were: increased accuracy of WRE risk assessments; improved audit case selection; increased risk detection; and reduced staff and resourcing costs. The substantiation risk model assesses the risk (likelihood and consequence) that the individual taxpayer’s WRE claims are non-compliant (case selection) and that each WRE claim is non-compliant (claim selection). During the design phase, the ATO noted improved case selection and increased revenue protection compared to the baseline nearest neighbour analysis model using 2015–2020 data. The selection of positive audit cases (those where there was non-compliance) improved by 19 percentage points (case selection model). The claim selection model increased non-compliant claim detections by an average of $475 per claim category across three WRE tax return labels. The ATO advised the ANAO on 15 July 2024 that potential model attributes for the substantiation risk model were selected ‘by business and data scientists based on the knowledge of the domain and available data’. The candidate models were assessed by data scientists, with the relevant business area confirming the models met their needs. The ATO also advised that analysis of data identified new attributes to be included in the candidate models, which were then included in the deployed risk model. |
Note a: ATO website, Latest estimates and trends.
Ethical AI assessments, including privacy and legality
3.16 To help maintain public trust in the use of AI, Australia’s AI Ethics Principles should be applied throughout the AI system lifecycle to support the ethical design, development and use of AI, including by respecting and upholding rights and data protection.109 The ATO’s six data ethics principles ‘outline the minimum standards that must be considered’ when conducting data or related activities to ‘address the main identified ethical risks that may arise’. AI can present additional privacy and legal risks. Case study 2 discusses ethics assessments, including assessments of privacy and legality, for the WRE AI models.
Case study 2. Data ethics assessments (including privacy and legality) for the WRE AI models |
Data ethics assessments Until December 2024, the ATO had not completed data ethics assessments for the WRE AI models, as required. Ethics assessments identify potential ethics risks, which should then be monitored once AI models are in operation. Without ethics assessments, the ATO was unable to sufficiently demonstrate that the WRE AI models were meeting ethical principles. Aspects of the WRE AI models were included in the pilot of the model ethics assessment process (discussed at paragraph 2.81). There was communication between the ATO’s data ethics team and the data science team (both within Smarter Data) related to the completion of a model ethics assessment as part of the pilot. The data ethics team requested updates in December 2023 and February 2024 with no response from the data science team. The ATO advised the ANAO in July 2024 that ‘[t]he WRE AI models are complex as they are incorporated into the broader business strategy in the Individuals and Intermediaries (IAI) Business Line beyond the WRE component’. This highlights a potential challenge in terms of operationalising AI ethics principles into a business context. The ATO completed a data ethics assessment for the WRE AI models in December 2024. It expects to complete a model ethics assessment in early 2025. Privacy and legality The ATO’s data ethics framework requires that AI models ‘uphold privacy, security and legality’.
|
Has the ATO established effective arrangements for the development of artificial intelligence models?
The ATO has not established a framework of policies and procedures for the development of AI models, although model development is to be documented in a standardised modelling solution report. For the 14 models that the ATO deployed between 1 July 2023 and 14 May 2024, there were differences in approaches as to: how data suitability was assessed and documented within the context of each model; how testing and validation was conducted; and decision-making arrangements for the development phase. For the work-related expenses AI models, the ATO assessed two potential biases. There was a lack of evidence to demonstrate that the ATO had considered whether data was fit for purpose and documented considerations relating to reproducibility.
Policies and procedures
3.17 The ATO has not developed a framework, including policies and procedures, to support the development of its AI models.110 The ATO advised the ANAO on 10 May 2024 that for model development:
Data Science teams design, build, evaluate and document their models. Version Control is through GitLab.111 Models are documented using the AP455 Modelling Solution Report, which is part of the ATO IT Delivery Method documentation suite.
3.18 Box 6 sets out the components of the standardised modelling solution reports (AP455s) that relate to the development of AI models.
Box 6: Aspects of the AP455 modelling solution report relating to model development |
|
Note a: Assumptions refer to the source currency, accuracy and if there are any missing fields.
Source: ATO documentation.
3.19 The ATO’s approach to developing models does not include the following.
- While the ATO has data governance and data management arrangements that relate to data quality, the ATO does not have a process for documenting whether data is fit for purpose for specific AI models. There was also a lack of documented process regarding reproducibility of model outputs and the testing and validation of models.112
- The ATO does not have a standard approach for controls to segregate duties in such a way that changes to AI models are adequately reviewed before being deployed. The ANAO observed instances where ATO officers made changes to code and reviewed their own changes.113 These control deficiencies extend to the approval and version control of AP455s which in some instances can be edited by ATO officers with edit access to the project without requiring approval.
Developing models in practice
3.20 The ANAO examined the development practices for the 14 AI models deployed between 1 July 2023 and 14 May 2024. Analysis identified that there was scope to better define considerations relating to data, bias and fairness, peer review and finalisation and approval. Table 3.3 provides a summary of the ANAO’s assessment (as of June 2024).
Table 3.3: Assessment of the development of the ATO’s AI models
Componenta |
ANAO assessment |
Key responsibilities |
Roles and responsibilities should include key personnel from both business and data science. Roles for the development phase were defined in PL191s, AP455s, and project and stakeholder engagement and communication plans. Responsibilities were not clearly defined for specified roles. |
Assessing whether data is fit for purpose |
|
Bias and fairness |
For the 14 models, the ATO did not provide evidence that it had assessed bias and fairness during the development phase. |
Reproducibility |
For the 14 models, current and historical versions of the code are stored in GitLab. Reproducibility is reliant on the availability of data from the relevant point in time. The ATO had not sufficiently documented reproducibility considerations for its AI models. |
Peer review and assurance |
For the 14 models, there was not a documented approach to peer review and assurance. Peer review and assurance included the following.
|
Finalisation and approval of the development phase |
The ATO advised the ANAO on 10 May 2024 that AP455s are used to document models. For 13 (93%) of the 14 models, the ATO referenced PL191s as providing evidence of the approval of the completion of the development phase. One referenced the AP455. The AP455s for the 14 models do not have documented approval. |
Note a: The components in this table were informed by: the AS ISO/IEC 42001:2023 Information technology — Artificial intelligence — Management system standard; Australia’s AI Ethics Framework; and the National framework for the assurance of artificial intelligence in government.
Source: ANAO analysis of ATO documentation.
Work-related expenses artificial intelligence models
3.21 The ANAO examined aspects of the development of the WRE AI models including: considerations of bias and fairness; and data quality, reliability and reproducibility.
Considerations of bias and fairness
3.22 Australia’s AI Ethics Principles include three principles relating to bias and fairness. These are: human, societal and environmental wellbeing (principle 1); human-centred values (principle 2); and fairness (principle 3).114 The National Institute of Standards and Technology states that:
As model implementation progresses and is trained on selected data, the effectiveness of bias mitigation should be evaluated and adjusted. During development, the organization [sic] should periodically assess the completeness of bias identification processes as well as the effectiveness of mitigation.115
3.23 Case study 3 outlines the ATO’s consideration of bias and fairness for the WRE AI models.
Case study 3. Bias and fairness consideration for the WRE AI models |
The ATO’s data ethics framework requires that ATO officers consider bias and fairness. Bias and fairness considerations are to be assessed as part of the following ATO data ethics principles: act in the public interest, be mindful of the individual; uphold privacy, security and legality; and engage in purposeful data activities. In completing data ethics assessments, ATO officers are to consider the risk of unfair biases arising from the use of AI. ATO guidance specifies that ‘unfair bias can occur through not only human decision-making, but the use of algorithms too’. ATO officers also need to consider limitations of the data, and the guidance states that ‘consideration needs to be given to biases, and mechanisms put in place to mitigate the continuation or creation of bias’. In December 2024, the ATO completed a data ethics assessment for the WRE AI models (see case study 2). Data ethics assessments were not mandated at the time of the development of the substantiation risk and the real-time analytics models. For the substantiation risk model, the ATO assessed two potential biases.
|
Data quality and reliability and model reproducibility
3.24 According to the National framework for the assurance of artificial intelligence in government, ‘the quality of an AI model’s output is driven by the quality of its data’.116 The International Organization of Supreme Audit Institutions states that ‘data quality and reliability is central to the performance of [machine learning] models’ and that ‘reproducibility [is] a mandatory condition for reliability’.117 Reproducibility is the ability to reproduce the model and model outcomes. Reproducibility includes the retention of data sources for reuse.118
3.25 Case study 4 outlines data and reproducibility considerations in the development of the WRE AI models.
Case study 4. Data quality and reliability, and model reproducibility — WRE AI models |
For the WRE AI modelsa, the ATO described: the data sources for the models; the populations; data processing, extraction and assembly; and data assumptions. The ATO conducted analysis on the quality and reliability of the data used for the models. An assessment of whether the data selected for the models was fit for purpose was not documented. In relation to the reproducibility, historical versions of code are located in GitLab. This supports reproducibility where relevant data remains available. The ATO did not document:
These issues mean that while a result may be able to be reproduced from a technical perspective, the ATO’s ability to explain the rationale for that result may be limited. The ATO advised the ANAO on 5 July 2024 that considerations for reproducibility are not documented, and that there is a ‘balance’ between keeping data for reproducibility purposes, and not wanting to keep data that is no longer needed. The ATO also advised that reproducibility can be impacted if systems used to develop and run models are decommissioned. |
Note a: The document understanding model is not included in this assessment as it was not in production.
Has the ATO established effective arrangements for the deployment of artificial intelligence models?
The ATO’s IT change enablement policy applies to all IT changes at the ATO, including the deployment of its AI models. For the 14 models that the ATO deployed between 1 July 2023 and 14 May 2024, practices varied for defining deployment criteria and deploying models. The ATO planned for model deployment and partially conducted verification and validation of models.
Policies and procedures
3.26 The ATO has not developed a specific framework, including policies and processes, for deploying its AI models. The ATO advised the ANAO in May and July 2024 the steps it takes to deploy AI models. Key aspects are set out in Box 7.
Box 7: Advice from the ATO about its approach to deploying AI models |
|
Source: ATO advice and documentation.
3.27 In addition to the advice set out in Box 7, the ATO’s IT change enablement policy applies to all applications, systems or services and to all production, test and development environments. The policy aims to ensure that all IT changes are implemented, managed and governed under a structured framework. This policy is supported by process guidance for deploying models.
3.28 The ANAO reviewed the ATO’s advice about deployment against relevant standards, frameworks, guidelines and policies and observed the following. The ATO’s advice does not specify a deployment planning process, including establishing technical performance criteria to be met prior to deployment.119 Without pre-defined performance criteria, the ATO’s processes do not establish when models should continue in production, be decommissioned or be re-deployed.120
Deploying models in practice
3.29 The ANAO examined the deployment practices for the 14 AI models deployed between 1 July 2023 and 14 May 2024. Overall, the ANAO found that there was a lack of clearly defined criteria to be met prior to deployment and there were varying processes for deploying models. Table 3.4 provides a summary of the ANAO’s assessment (as of June 2024).
Table 3.4: Assessment of the deployment of ATO’s AI models
Componenta |
ANAO assessment |
Planning for deployment |
Planning for deployment is documented in AP455s — each of the 14 models had an AP455. Of these, 12 (86%) provided evidence of planning for deployment by demonstrating consideration of deployment criteria, verification and validation measures, testing, and stakeholder consultation. |
Business verification, validation and approval |
Business verification and validation involves comparing planned and actual performance in production.
Business approval is required prior to deployment. Approval from business was documented for all 14 models. The ATO advised that models may have a business deployment verification after deployment. There was no evidence of this verification for any of the 14 models. |
Technical verification, validation and approval |
Technical verification and validation involve approval by the model developers that the models are ready to be deployed.
|
Deployment and change management |
IT changes can be categorised as standard, normal or emergency under the ATO’s IT change enablement policy. The ATO advised the ANAO on 16 September 2024 that the 14 AI models were deployed as standard changes.b
The ATO’s IT change enablement policy requires demonstration of segregation of duties. The ATO did not undertake segregation of dutiesc for deployment tasks in two (14%) of the 14 models. |
Note a: The components in this table were informed by AS ISO/IEC 2001:2023 Information technology — Artificial intelligence — Management system, and the ATO’s IT change enablement policy.
Note b: A standard change is a low-risk, predictable and repeatable change.
Note c: Segregation of duties requires that developers building and testing software cannot have a role in deploying or promoting their own code to production environments.
Source: ANAO analysis of ATO documentation.
Recommendation no.5
3.30 The ATO develop and implement policies and procedures to support the effective design, development, deployment and assurance of AI models. Where relevant ATO policies and procedures exist, the ATO ensure that the design, development and deployment of AI models aligns with these.
Australian Taxation Office response: Agreed.
3.31 The ATO will review and where necessary develop policies, procedures and associated guidance to support the effective delivery of AI models.
4. Monitoring, evaluating and reporting on the adoption of artificial intelligence
Areas examined
This chapter examines whether the Australian Taxation Office (ATO) is effectively monitoring, evaluating and reporting on its adoption of artificial intelligence (AI).
Conclusion
The ATO has partly effective arrangements to monitor, evaluate and report on its adoption of AI.
- There was no evidence of structured and regular monitoring of ATO-built AI models in production. This was being addressed through the development of a monitoring and reporting framework for its AI models.
- The ATO did not regularly report on the implementation of its automation and AI strategy between October 2022 and January 2024. It has developed a monthly report since February 2024 to report on the implementation of the strategy. It has not set out an evaluation approach for the strategy.
Areas for improvement
The ANAO made two recommendations aimed at: establishing performance measurement and evaluation arrangements for the automation and AI strategy; and ensuring that the management of information supports transparency and accountability with respect to the adoption of AI.
The ANAO suggested that the ATO could monitor outcomes and unintended impacts of using AI and introduce a more structured approach to continuous improvement.
4.1 Entities should document AI objectives121 and ‘commit to implementing robust AI system performance monitoring and evaluation, and to ensuring each system remains fit for purpose’.122 Evaluation helps to ‘confirm that expected results are being achieved and risks are being managed’.123
4.2 Entities should also monitor and evaluate the performance and effectiveness of its arrangements for managing AI. This includes setting out: what needs to be monitored and evaluated; the methods and timing for monitoring and evaluation; and how results will be documented.124
Is the ATO effectively monitoring, evaluating and reporting on the implementation of artificial intelligence models?
The ATO does not have policies and procedures supporting the monitoring and evaluation of its in-house built AI models. For the 14 AI models built and deployed between 1 July 2023 and 14 May 2024, there was no evidence of ongoing performance monitoring and reporting. For the work-related expenses AI models, there were some examples of monitoring and evaluation. Baselines were not reported in ongoing monitoring and reporting to show the impact of introducing the models.
Policies and procedures
4.3 The ATO has not developed a framework of policies and processes to support monitoring, evaluation and reporting for its AI models. The ATO advised the ANAO on 10 May 2024 and 3 July 2024 of the steps it undertakes in the ‘execution and monitoring’ and ‘decommissioning’ phases (see Box 8).
Box 8: Advice from the ATO about its approach to monitoring AI models |
|
Note a: The ATO advised the ANAO in December 2024 that it decommissioned two AI models between 1 July 2023 and 14 May 2024.
4.4 The ANAO compared the ATO’s advice with relevant standards, frameworks, guidelines and policies.
- The ATO’s approach does not include developing model operating and monitoring plans for system repairs, updates, and support and corrective actions.125
- The ATO does not have processes for the assessment of the ongoing utility of an AI system, including for model drift126, which is a key risk to model reliability and safety.127 It does not have a defined approach to setting out the measures which should lead to model changes (such as retraining) or decommissioning.
- The ATO does not have a standardised approach for establishing performance metrics and benchmarks.128 The ATO is developing a performance metrics library under its automation and AI strategy (A&AI strategy).
- The ATO does not have a consistent approach for training ATO officers who use AI.129
4.5 In May 2024, the ATO issued a direction to staff in relation to: implementing peer review; regular performance monitoring; model refresh; and six-monthly review cycles. In relation to this direction, the ATO developed guidance for reviewing models prior to deployment, and created arrangements to support performance monitoring and review of models.
Monitoring and evaluation of models in practice
4.6 The ANAO examined the monitoring and evaluation practices for the 14 AI models deployed between 1 July 2023 and 14 May 2024. Overall, the ANAO found there was a lack of monitoring and evaluation. Table 4.1 provides a summary of the ANAO’s assessment (as of June 2024).
Table 4.1: Assessment of the monitoring of the ATO’s AI models
Componenta |
ANAO assessment |
Model performance |
Performance monitoring includes monitoring of technical model metrics and monitoring of the impact of the model on business outcomes.
|
Issues logging and management |
The ATO did not have a documented process for the development of operating and monitoring plans, identifying issues following model deployment, and undertaking corrective actions. For one (7%) of 14 models, the ATO set out aspects of ongoing maintenance considerations in design documentation, including description of a model continuous learning pipeline, and definition of retraining triggers based on performance metrics. This design document was not approved. Evidence of this type of planning was not evident in the other models deployed. |
Training |
There was no standard approach to training for business users of AI models. For one (7%) of the 14 models, the ATO developed specific training materials for model users. The ATO advised that for 10 (71%) of the 14 models, training was not required for users due to users’ pre-existing knowledge. |
Monitoring of ethical considerations |
The 14 models were not monitored on an ongoing basis for reliability, fairness and other ethical considerations. |
Evaluation and benefits realisation |
The ATO did not have an evaluation approach.c Of the 14 models, one had completed closure documentation and an evaluation; eight had completed closure documentation and no evaluation; and five models had no closure documentation and no evidence of evaluation. |
Note a: The components in this table were informed by: AS ISO/IEC 42001:2023 Information Technology — Artificial intelligence — Management system; Australia’s AI Ethics Principles and the ATO’s Benefits Management Framework.
Note b: Unsupervised learning uses machine learning algorithms to analyse unlabelled data sets.
Note c: At the time of this assessment (June 2024), all models had been in production for less than 12 months.
Source: ANAO analysis of ATO documentation.
4.7 The Policy for the responsible use of AI in government outlines that entities should monitor AI use cases to assess for unintended impacts. The policy also requires that entities publish AI transparency statements by 28 February 2025 that include ‘information on measures to monitor [the] effectiveness of deployed AI systems’.130
Opportunity for improvement
4.8 The ATO could ensure that monitoring and evaluation arrangements assess each model in relation to the achievement of business outcomes and monitor for unintended impacts.
Work-related expenses artificial intelligence models — benefits and outcomes
4.9 The ANAO examined the ATO’s monitoring and evaluation of the work-related expenses (WRE) AI models (see Appendix 4). Case study 5 describes the ATO’s process of defining benefits and measuring outcomes for the models.
Case study 5. Measuring the outcomes of the WRE AI models |
The substantiation risk model aims to contribute to the ATO’s approach to reduce the tax gap by increasing accuracy of WRE risk assessments identified for review by ATO officers. For this model, outcome measures reported include financial results which measure reductions in WRE claims identified by the models and strike rates. Strike rates are defined as ‘the percentage of closed cases that have an outcome where outcome refers to one or more claims having been adjusted after review’. As of July 2024, strike rates reported by the ATO for the two WRE AI models were 97.86 per cent (WRE substantiation model) and 99.60 per cent (WRE high risk self-preparers). The ATO advised the ANAO on 15 July 2024 that baselines were established prior to the introduction of the models in August 2021. Comparison against baseline measures is not included in the ATO’s ongoing reporting.a The intended benefits for the document understanding component of the WRE AI models are increased accuracy and faster processing times by case officers when undertaking an audit, as the document understanding component aims to identify and recommend to ATO officers the relevant information provided as WRE claims substantiation evidence by taxpayers. The document understanding component has undergone an extended pilot phase and has had multiple evaluations. The draft evaluation from Tax Time 2022 found that document understanding overall did not significantly reduce processing times. As of July 2024, further evaluations to measure processing times have not been completed as the pilot is continuing. |
Note a: Under the Commonwealth Evaluation Policy, entities are to ‘plan to conduct fit for purpose monitoring and evaluation activities before beginning any program or activity. This includes identifying time-frames, resources, baseline data and performance information’. Department of the Treasury, What is evaluation, Treasury, available from https://evaluation.treasury.gov.au/toolkit/what-evaluation [accessed 23 October 2024].
Is the ATO effectively monitoring, evaluating, and reporting on the enterprise-wide adoption of artificial intelligence?
The ATO has a project underway to introduce an enterprise-wide approach to monitoring the performance of its AI models by December 2026. The ATO has developed a ‘use of publicly available generative AI technology policy’. The ATO does not report on compliance with this policy to relevant internal governance bodies. For its automation and AI strategy, the ATO introduced status reporting in February 2024. It does not have arrangements in place to measure the effectiveness of the strategy. Some continuous improvement arrangements were evident including an internal governance review and the delivery of the automation and AI strategy. There is a need for the ATO to improve its management of information in support of the transparent and accountable adoption of AI.
Enterprise-wide monitoring and evaluation of the adoption of AI
4.10 As outlined in Chapter 2, the ATO is developing AI-specific governance arrangements including an enterprise AI policy and AI risk assessment. The ATO’s desired ‘future state’ for AI governance recognises the need for additional monitoring, evaluation and reporting.
Monitoring the use of AI
4.11 The ATO does not have centralised and standardised monitoring and reporting arrangements relating to its use of AI. Under use case five of the A&AI strategy, the ATO is developing arrangements to support a consistent approach to the monitoring and evaluation of its AI models and other data and analytics models.
4.12 Planning documents for use case five (enterprise learning feedback loop) outline that ‘there is no centralised and maintained repository of model metadata, or registry models in production’ (see Table 2.2). As such, it involves manual, ‘costly and time intensive’ processes to extract information about models. Further, the lack of a centralised registry ‘presents itself during the model maintenance phase where models may not have clearly defined performance monitoring structures established. It becomes difficult to assess which models are operating effectively, and which models need to be retrained or retired’.
4.13 Full implementation of the model register and performance monitoring components of use case five is planned for December 2026.
Use of publicly available generative AI
4.14 Under the ATO’s ‘use of publicly available generative AI technology policy’, ATO officers are required to comply with a range of ATO policies, such as policies on data management; privacy and confidentiality; proper use of information technology equipment; security; and social media.
4.15 The Generative AI SES Band 1 Working Group and Generative AI Band 2 Group are to monitor and report on the policy, including any breaches of the policy. The Generative AI Band 1 Working Group met 17 times between 15 September 2023 and August 2024. The Generative AI SES Band 2 Working Group met nine times between 28 September 2023 and August 2024. Both groups review applications and associated risk assessments from ATO officers to use publicly available generative AI tools. There was no reporting to these governance bodies in relation to compliance (or non-compliance) with the ‘use of publicly available generative AI technology policy’.
4.16 The ATO advised the ANAO that more than 2000 ATO staff are using approved generative AI tools. As of December 2024, six breaches of the ATO’s generative AI policy had been reported — all six breaches were self-reported by the relevant officer. The six breaches involved the unauthorised use of a generative AI technology (either an unapproved tool was used, or an approved tool was used in an unapproved way).
Artificial intelligence strategy
4.17 The ATO’s A&AI strategy was approved in October 2022 and is focussed on the delivery of five enterprise A&AI use cases (see paragraphs 2.19 to 2.28).
Monitoring implementation and reporting
4.18 Between October 2022 and January 2024, there was no regular internal monitoring and reporting on the implementation of the A&AI strategy.
4.19 In February 2024, the ATO developed a monthly report to monitor the implementation of the five use cases of the A&AI strategy. The reports are provided to the Deputy Commissioner Smarter Data, and relevant Senior Executive Service Band 1 officers across the ATO. Table 4.2 provides a summary of the status of the five A&AI use cases as at June 2024.
Table 4.2: Reporting status of five A&AI strategy use cases, as at June 2024
Use case |
Reporting statusa |
|
1a. Document understanding — risk treatment |
Amber |
Risks reported for the delivery of this use case included resourcing constraints and uncertain ongoing future funding. |
1b. Document understanding — intelligence |
Green |
The project for this use case was reported as ‘green’, with research being conducted to scope the project. Risks reported for the delivery of this use case included uncertain ongoing future funding and delays in obtaining required data. |
2. Risk and fraud identification |
Green |
The ATO reported this use case as ‘green’ as the delivery of the first-year tranche of work was on track to be delivered as planned. It also reported a risk related to uncertain ongoing future funding. |
3. Integrated profiling of client |
Amber |
The ATO reported that the delivery of this use case was being impacted by the lack of dedicated resourcing. |
4. Assisted law interpretation and insight |
Green |
The ATO reported this use case as ‘green’, with proof of concepts developed. Key risks reported include insufficient resourcing, uncertain funding arrangements and delays in obtaining the appropriate security risk assessment. |
5. Enterprise learning feedback loop |
Amber |
The ATO reported that the delivery of this use case is being impacted by the lack of resourcing with required skillsets and funding pressures. |
Note a: Green means ‘on track’; amber means that ‘impacts/mitigations are under review’; and red means ‘action required’.
Source: ATO documentation.
Measurement and evaluation
4.20 The vision of the A&AI strategy is that ‘by 2030 the ATO is a leader in developing and industrialising ethical, impactful and scalable A&AI solutions, creating immense economic and social benefit for our nation and citizens’. The A&AI strategy includes five statements of success:
- AI ethics is at the heart of everything;
- there is enterprise-wide client personalisation;
- there is auto content extraction and understanding for most documents;
- pre-emptive action and real-time interaction is the norm; and
- human and AI are interacting partners through feedback integration and Application Programming Interfaces.
4.21 The ATO has not developed an evaluation approach against the A&AI strategy.
Recommendation no.6
4.22 The ATO establish performance measurement and evaluation arrangements for its automation and AI strategy.
Australian Taxation Office response: Agreed.
4.23 The ATO will establish performance measurement and evaluation arrangements for its automation and AI strategy.
Related strategies and business plans
4.24 As outlined at paragraph 2.22, the ATO has other strategies and plans that include components relating to AI. The ATO does not have overarching monitoring and reporting frameworks for its digital strategy and its data and analytics strategy.
Continuous improvement
4.25 The AS ISO/IEC 42001:2023 Information technology — Artificial intelligence — Management system standard highlights that organisations should ‘continually improve the suitability, adequacy and effectiveness of the AI management system’.131 The Policy for the responsible use of AI in government states that entities should review ‘on an ongoing basis the internal policies and governance approaches to AI to ensure they remain fit for purpose’.132
4.26 The ATO did not have a structured approach to continuous improvement, although improvement activities had occurred. Activities include: an ATO Executive initiated review of AI governance — this was closed without being finalised (November 2022); an internal paper on the application of the ATO’s data governance and management arrangements to AI (April 2024); and that continuous improvement forms part of the A&AI strategy.
4.27 Internal audits can form part of an entity’s continuous improvement arrangements. Between 1 July 2022 and 12 March 2024, the ATO did not undertake any internal audits on its enterprise-wide adoption of AI. The ATO’s internal audit team reported to the ATO’s Audit and Risk Committee in March 2022 that it had provided guidance to Smarter Data on appropriate governance for AI, and that it had identified gaps in the ATO’s AI governance arrangements. The ATO was unable to locate this advice.
Opportunity for improvement
4.28 The ATO could develop a more structured approach to continuous improvement in relation to its adoption of AI.
Information management
4.29 The management of information is fundamental to sound public sector administration.133 The National Archives of Australia has noted that record keeping obligations are ‘technology and format neutral’ and that records in relation to AI should document research, development, use and management.134 Maintaining reliable data and information assets is important for the development and maintenance of ethical AI by supporting transparency, explainability and accountability.135
4.30 The ANAO identified information management issues throughout the course of the audit including poor records of: governance, planning and oversight; risk management; activities throughout the AI system lifecycle; and key decisions. These issues have impacted the ability of the ATO to demonstrate transparency and accountability with respect to its adoption of AI.136 Addressing these issues will be important to support additional transparency requirements and expectations, such as the requirement to produce transparency statements as part of the Policy for the responsible use of AI in government.
Recommendation no.7
4.31 The ATO ensure that its approach to managing information supports transparency and accountability with respect to its adoption of AI.
Australian Taxation Office response: Agreed.
4.32 The ATO will ensure the approach to record keeping and documentation supports transparency and accountability with respect to the adoption of AI.
Appendices
Appendix 1 Entity response
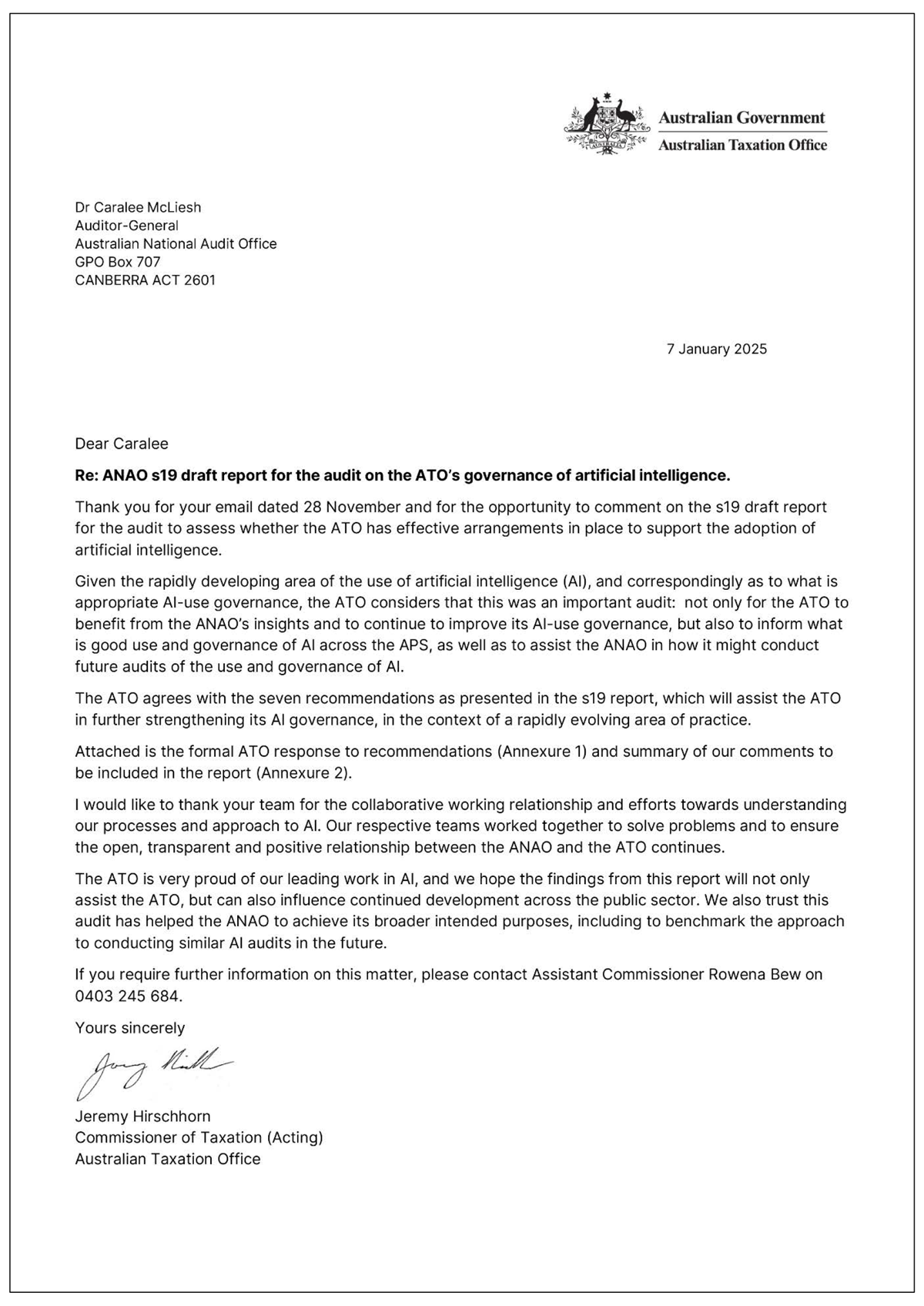
Appendix 2 Improvements observed by the ANAO
1. The existence of independent external audit, and the accompanying potential for scrutiny improves performance. Improvements in administrative and management practices usually occur: in anticipation of ANAO audit activity; during an audit engagement; as interim findings are made; and/or after the audit has been completed and formal findings are communicated.
2. The Joint Committee of Public Accounts and Audit (JCPAA) has encouraged the ANAO to consider ways in which the ANAO could capture and describe some of these impacts. The ANAO’s Corporate Plan states that the ANAO’s annual performance statements will provide a narrative that will consider, amongst other matters, analysis of key improvements made by entities during a performance audit process based on information included in tabled performance audit reports.
3. Performance audits involve close engagement between the ANAO and the audited entity as well as other stakeholders involved in the program or activity being audited. Throughout the audit engagement, the ANAO outlines to the entity the preliminary audit findings, conclusions and potential audit recommendations. This ensures that final recommendations are appropriately targeted and encourages entities to take early remedial action on any identified matters during the course of an audit. Remedial actions entities may take during the audit include:
- strengthening governance arrangements;
- introducing or revising policies, strategies, guidelines or administrative processes; and
- initiating reviews or investigations.
4. In this context, the below actions were observed by the ANAO during the course of the audit. It is not clear whether these actions and/or the timing of these actions were planned in response to proposed or actual audit activity. The ANAO has not sought to obtain assurance over the source of these actions or whether they have been appropriately implemented.
- The ATO was developing an enterprise-wide AI policy and AI risk management guidance (paragraph 2.5).
- The ATO updated its data ethics framework to incorporate a model ethics assessment process (paragraph 2.5).
- In January 2024, the ATO established an AI Governance team within the Data Management branch in Smarter Data (paragraph 2.33).
- The ATO established a Data and Analytics Governance Committee (paragraph 2.38).
- The ATO reviewed its two AI-related risks in December 2024 (paragraph 2.58).
- The ATO completed a data ethics assessment for the work-related expenses AI models in December 2024 (case study 2).
- The ATO was developing a performance metrics library under its automation and AI strategy (paragraph 4.4).
- The ATO developed guidance for reviewing models prior to deployment, and created arrangements to support performance monitoring and review of models (paragraph 4.5).
- The ATO was developing a register and standardised performance monitoring and reporting framework for its AI models (paragraph 4.11).
- In February 2024, the ATO developed a monthly status report to monitor the implementation of the automation and AI strategy (paragraph 4.19).
Appendix 3 AI models deployed by the ATO between 1 July 2023 and 14 May 2024
1. The ATO deployed 14 AI models between 1 July 2023 and 14 May 2024. The ANAO assessed the design, development, deployment and monitoring of these models — a list of the models and their descriptions is provided in Table A.1.
Table A.1: AI models deployed by the ATO between 1 July 2023 and 14 May 2024
Model name |
Model description |
Black Economy Omitted Income Scoring Job |
This model aims to generate predictions on the likelihood and consequence of omitted income in the small business market. These predictions can then be used as guidance on newly opened compliance cases. |
Capital Gain Tax Modelling |
This model predicts the likelihood that property sold in the prior week was the taxpayer’s main residence and therefore eligible for various legislative exemptions from capital gains tax. |
Rental Risk Modelling |
This model aims to assess interactions and ratios between rental incomes and expenses to find patterns of non-compliance. It aims to improve the strike rate by learning from past audit outcomes to allow better allocation of resources. |
Revenue on Disposal of Real Property model |
This model aims to identify clients at risk of having misclassified their disposal of real property as capital rather than revenue. It assigns a predictive likelihood and consequence value for each client. |
Tax Practitioner Risk Model (TPRM) Goods and Services Tax (GST) Stratified Sampling |
This model examines whether the amount of GST reported by tax practitioners for their clients is accurate, by comparing the amounts to other tax agents. The model scope includes the GST of sales and the GST of purchases, focusing on whether the amount is understated. |
TPRM Liability Model Stratified Sampling |
This model aims to assess tax agents on their client’s incidence of omitted income in comparison to other tax agents. This is assessed for both business and non-business income. |
TPRM Income Tax Return Lodgment |
This model aims to identify the level of influence a tax agent has over the lodgment performance for their clients’ income tax returns. The model can predict and classifies a taxpayer on whether they will lodge on time, and then compares the information on lodgment compliance to other tax agents. |
TPRM Business Activity Statement Lodgment |
This model aims to identify the level of influence a tax agent has over their client’s lodgment performance of business activity statements. The model ranks tax agents by their influence over lodgment performance and the incidence of their client’s lodgment compliance. |
TPRM Pattern Tests |
This model aims to identify patterns in tax agent behaviour relating to reporting behaviour. |
TPRM Pay as You Go Withholding |
This model aims to provide an agent view of risk for employer compliance and identify the influence tax agents have on the employer’s compliance. |
TPRM Rental Property Expenses |
This model aims to assess tax agents by comparing their clients’ total rental expenses to other tax agent clients. This includes assessing rental property expenses both including purchase price and excluding purchase price. |
TPRM Small Business Stratified Sampling |
This model aims to assess whether a tax agent is understating the amount of total business income their clients reported to the ATO or overstating total deductible expenses which are claimed by the taxpayer. |
TPRM Superannuation Guarantee |
This model aims to provide the ATO with an agent level view of superannuation guarantee obligations to assist in identifying and treating tax agents who pose a risk to client compliance. |
TPRM Unexplained Wealth |
This model aims to assess tax agents on the incidence and the amount of their clients’ household lifestyle income (either shortfall or surplus) in comparison to other tax agents. |
Source: ATO documentation.
Appendix 4 Overview of the ATO’s work-related expenses AI models
1. Table A.2 provides an overview of the ATO’s four AI models in use for work-related expenses (WRE) claims.
Table A.2: WRE AI models, as of 14 May 2024
WRE AI model and description |
Deployed |
1. Substantiation risk model |
|
The ATO’s substantiation risk model assesses the risk (likelihood, severity and consequence) that WRE expenses claims are not compliant with legislation and ATO policy. It includes two components:
|
5 August 2021 |
2. Nexusa |
|
Nexus incorporates two models which are used to evaluate the likelihood that a taxpayer’s WRE claims directly relate to their occupation:
|
24 June 2022b |
3. Document understanding |
|
The document understanding component aims to use computer vision and natural language processing to understand documentation and evidence provided to support WRE claims. The ATO first started using the document understanding models in 2021 as a pilot. The ATO advised the ANAO on 22 March 2024 that they have not yet been deployed into production as planned due to insufficient graphic processing unit (GPU) hardware and software capacity. Research has been underway to modify its architecture to expand its usage. |
Not yet in production |
4. Real-time analytics |
|
The real-time analytics component involves nearest neighbour analysis (NNA) to assess the claims that the ATO expects similar taxpayers to make.c NNA is used to assess WRE risk at the time of lodgment. All components of the substantiation risk model use different variants of NNA, for example, to inform risk consequence and likelihood scores through comparison of other, similar taxpayer claims. |
2016 |
Note a: Nexus refers to the requirement for taxpayer’s WRE claims to directly relate to their occupation.
Note b: The ATO advised the ANAO on 16 September 2024 that ‘WRE Nexus was ‘productionised’ in June 2022 to enable two case officers to view the outputs and provide verbal feedback to the technical team. It has not been used to inform or determine case outcomes’.
Note c: NNA assumes the average behaviour of a population is compliant.
Source: ANAO analysis of ATO documentation.
Appendix 5 Audit methodology — key sources
1. This audit assessed the ATO’s governance arrangements supporting the adoption of AI — with a focus at both the organisational level and the AI system level.
2. The methodology for this audit was informed by a range of sources from: the Australian Government sector; other Australian jurisdictions; and frameworks from other jurisdictions. Throughout the audit, references are specified where relevant. Table A.3 lists the key sources that underpinned the ANAO’s methodology.
Table A.3: Sources supporting the ANAO’s methodology for this audit
Category |
Source |
Australian Government — AI specific |
|
Australian legislation |
|
Other Australian Government policies and frameworks — non-AI specific |
|
Other Australian — AI specific |
|
International — AI specific |
|
Source: As specified in table.
Appendix 6 AI system lifecycles
1. The following figures present depictions of AI system lifecycles — one from the Organisation for Economic Co-operation and Development and the other from the Government Accountability Office.
Figure A.1: AI system lifecycle — Organisation for Economic Co-operation and Development

Source: Organisation for Economic Co-operation and Development, OECD AI Principles overview, OECD, available from https://oecd.ai/en/ai-principles [accessed 21 October 2024].
Figure A.2: AI system lifecycle — Government Accountability Office
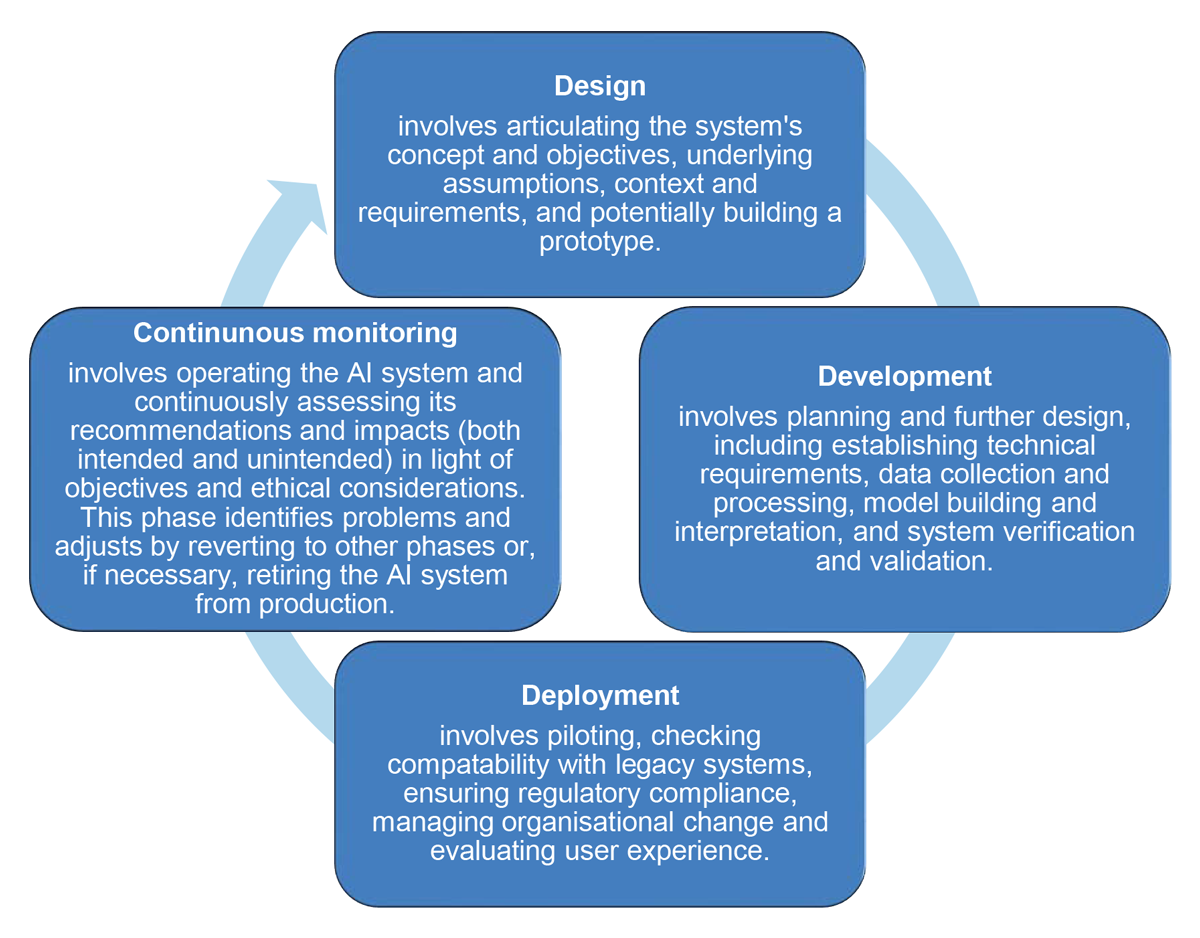
Source: Government Accountability Office, GAO-21-519SP Artificial Intelligence Accountability Framework, GAO, p. 17, available from https://www.gao.gov/assets/gao-21-519sp.pdf [accessed 2 June 2024].
Footnotes
1 Parliament of Australia, Senate Select Committee on Adopting Artificial Intelligence, available from https://www.aph.gov.au/Parliamentary_Business/Committees/Senate/Adopting_Artificial_Intelligence_AI/AdoptingAI [accessed 18 February 2025].
2 Parliament of Australia, Inquiry into the use and governance of artificial intelligence systems by public sector entities, available from https://www.aph.gov.au/Parliamentary_Business/Committees/Joint/Public_Accounts_and_Audit/PublicsectoruseofAI [accessed 18 February 2025].
3 OECD, Explanatory memorandum on the updated OECD definition of an AI system, OECD, March 2024, p. 4, available from https://www.oecd-ilibrary.org/science-and-technology/explanatory-memorandum-on-the-updated-oecd-definition-of-an-ai-system_623da898-en [accessed 5 April 2024].
4 Australian Government, Data and Digital Government Strategy, 15 December 2023, pp. 16 and 20, available from https://www.dataanddigital.gov.au/ [accessed 5 April 2024].
5 Australian Government et al., National framework for the assurance of artificial intelligence in government, Australian Government, 21 June 2024, available from https://www.finance.gov.au/government/public-data/data-and-digital-ministers-meeting/national-framework-assurance-artificial-intelligence-government [accessed 26 June 2024].
6 Digital Transformation Agency, Policy for the responsible use of AI in government, DTA, August 2024, available from https://www.digital.gov.au/policy/ai/policy [accessed 16 August 2024].
7 The ATO defines AI models as: algorithms designed to mimic or surpass human intelligence and make predictions based on data; and that use mathematical, statistical or machine learning techniques trained on extensive datasets to process and analyse information. This audit report uses the term ‘AI models’ when referring to the AI models built in house by the ATO.
8 This audit includes an examination of the ATO’s AI models that it uses in the context of processing and assessing work-related expenses claims — see Appendix 4 for a list and description of these models.
9 Department of the Prime Minister and Cabinet, Australian Government Long-term Insights Briefing — How might artificial intelligence affect the trustworthiness of public service delivery?, PM&C, 2023, pp. 6–7 available from https://www.pmc.gov.au/resources/long-term-insights-briefings/how-might-ai-affect-trust-public-service-delivery [accessed 18 January 2024].
10 Digital Transformation Agency, Adoption of Artificial Intelligence in the Public Sector, DTA, available from https://architecture.digital.gov.au/adoption-artificial-intelligence-public-sector [accessed 15 July 2024].
11 Organisation for Economic Co-operation and Development, OECD Framework for the Classification of AI Systems, OECD, February 2022, p. 3, available from https://www.oecd.org/publications/oecd-framework-for-the-classification-of-ai-systems-cb6d9eca-en.htm [accessed 19 May 2024].
12 ATO, How we use data and analytics, 2022, available from https://www.ato.gov.au/about-ato/commitments-and-reporting/information-and-privacy/how-we-use-data-and-analytics [accessed 12 March 2024].
13 The OECD has noted the difficulty in obtaining a consensus on a definition for AI systems and that if governments are to ‘legislate and regulate AI, they need a definition as a foundation’. OECD, Updates to the OECD’s definition of an AI system explained, OECD, available from https://oecd.ai/en/wonk/ai-system-definition-update [accessed 5 April 2024].
14 For example, email spam filters, which were once considered advanced AI, have become so widely utilised that people no longer view them as a form of AI. IBM Center for The Business of Government, Risk Management in the AI Era: Navigating the Opportunities and Challenges of AI Tools in the Public Sector, 15 April 2020, p. 18, available from https://www.businessofgovernment.org/report/risk-management-ai-era-navigating-opportunities-and-challenges-ai-tools-public-sector [accessed 4 September 2024].
15 Digital Transformation Agency, Policy for the responsible use of AI in government, DTA, August 2024, available from https://www.digital.gov.au/policy/ai/policy [accessed 16 August 2024].
16 Australian Institute of Company Directors and Human Technology Institute, A Director’s Guide to AI Governance, p. 10, available from https://www.aicd.com.au/content/dam/aicd/pdf/tools-resources/director-resources/a-directors-guide-to-ai-governance-web.pdf [accessed 29 August 2024].
17 Department of Industry, Science and Resources, Safe and responsible AI in Australia Consultation – Australian Government’s interim response, 2024, p. 4, available from https://www.industry.gov.au/news/australian-governments-interim-response-safe-and-responsible-ai-consultation [accessed 12 March 2024].
18 Australian Government, Data and Digital Government Strategy, pp. 16 and 20.
19 OECD.AI, OECD AI Principles overview, OECD.AI, 2024, available from https://oecd.ai/en/ai-principles [accessed 17 July 2024].
20 Department of Industry, Science and Resources, Australia’s AI Ethics Principles, DISR, 2019, available from https://www.industry.gov.au/publications/australias-artificial-intelligence-ethics-framework/australias-ai-ethics-principles [accessed 17 July 2024].
21 These principles were released in 2019 and updated in May 2024 to consider new technological and policy advancements.
22 Department of Industry, Science and Resources, Australia’s Artificial Intelligence Action Plan, DISR, 2021, available from https://www.industry.gov.au/publications/australias-artificial-intelligence-action-plan [accessed 25 July 2024].
23 Department of Industry, Science and Resources, Australia’s Artificial Intelligence Ethics Framework, DISR, 7 November 2019, available from https://www.industry.gov.au/publications/australias-artificial-intelligence-ethics-framework [accessed 5 April 2024].
24 Auditor-General Report No. 22 2024–25, Audits of the Financial Statements of Australian Government Entities for the Period Ended 30 June 2024, ANAO, Canberra, 2024, paragraphs 9 and 3.30, available from https://www.anao.gov.au/work/financial-statement-audit/audits-of-the-financial-statements-of-australian-government-entities-the-period-ended-30-june-2024 [accessed 12 February 2025].
25 Australian Government et al., National framework for the assurance of artificial intelligence in government, Australian Government, 21 June 2024, see ‘Statement from Data and Digital Ministers’, available from https://www.finance.gov.au/government/public-data/data-and-digital-ministers-meeting/national-framework-assurance-artificial-intelligence-government [accessed 26 June 2024].
26 ibid., ‘Introduction’.
27 Digital Transformation Agency, Policy for the responsible use of AI in government, DTA, August 2024, available from https://www.digital.gov.au/policy/ai/policy [accessed 16 August 2024].
28 The policy is mandatory for non-corporate Commonwealth entities, except for the Defence portfolio and the national intelligence community. Corporate Commonwealth entities are encouraged to apply the policy.
29 Accountable officials must: be accountable for implementation of the policy within their entities; notify the Digital Transformation Agency where the entity has identified a new high-risk use case; be a contact point for whole-of-government AI coordination; engage in whole-of-government AI forums and processes; and keep up to date with changing requirements.
30 This statement must provide the public with relevant information about the entity’s use of AI including information on: compliance with this policy; measures to monitor the effectiveness of deployed AI systems; and efforts to protect the public against negative impacts.
31 ATO, Corporate Plan 2024–25, 13 August 2024, available from https://www.ato.gov.au/about-ato/managing-the-tax-and-super-system/strategic-direction/corporate-plan [accessed 4 October 2024].
32 ATO, How we use data and analytics, 14 September, 2022, available from https://www.ato.gov.au/about-ato/commitments-and-reporting/information-and-privacy/how-we-use-data-and-analytics [accessed 12 March 2024].
33 ATO submission to the Joint Committee of Public Accounts and Audit’s inquiry into the Commonwealth financial statements, ’20.2 Supplementary to submission 20’, pp. 1–2, available from https://www.aph.gov.au/Parliamentary_Business/Committees/Joint/Public_Accounts_and_Audit/CFS2022-23/Submissions [accessed 11 November 2024].
34 Auditor-General Report No. 22 2024–25, Audits of the Financial Statements of Australian Government Entities for the Period Ended 30 June 2024, p. 10.
35 Australian Government et al., National framework for the assurance of artificial intelligence in government, Australian Government 21 June 2024, see ‘Governance’, available from https://www.finance.gov.au/government/public-data/data-and-digital-ministers-meeting/national-framework-assurance-artificial-intelligence-government [accessed 26 June 2024].
36 As outlined in paragraph 1.25, the scope of this audit does not include an assessment of the ATO’s data governance and data management more broadly.
37 The ATO’s data lifecycle includes four phases: source and create; store and manage; use and share; and archive and dispose.
38 The planned consultation process includes consultation with: internal subject matter experts; key internal stakeholder groups more broadly; key ATO governance committees and corporate functions; ATO staff and unions; and the ATO Executive.
39 Components assessed were: authorising framework; policies; guidelines; system controls; procedures; conformance; capability; and communications.
40 The assessment noted that maturity was assessed based on the Department of Finance’s Data Maturity Assessment Tool, available from https://www.finance.gov.au/government/public-data/public-data-policy/data-maturity-assessment-tool [accessed 23 July 2024].
41 Digital Transformation Agency, Policy for the responsible use of AI in government, DTA, August 2024, available from https://www.digital.gov.au/policy/ai/policy [accessed 16 August 2024].
42 Digital Transformation Agency, Interim guidance on government use of public generative AI tools, DTA, 22 November 2023, available from https://architecture.digital.gov.au/generative-ai [accessed 10 April 2024].
43 Prior this policy, the ATO had issued guidance to staff on the use of generative AI on 1 May 2023.
44 ATO submission to the Joint Committee of Public Accounts and Audit inquiry into the use and governance of artificial intelligence systems by public sector entities, October 2024, p. 3, available from https://www.aph.gov.au/Parliamentary_Business/Committees/Joint/Public_Accounts_and_Audit/PublicsectoruseofAI/Submissions [accessed 13 November 2024].
45 The ATO advised that the ‘main features [of the software] used to process and analyse data include indexing, Optical Character Recognition (OCR), indexed search capability, tagging document of relevance, email network visualisation and simple Named Entity Recognition’.
46 Australian Government et al., National framework for the assurance of artificial intelligence in government, Australian Government, ‘6. Transparency and explainability’.
47 ibid.
48 A model can have more than one type of automated action.
49 A nudge is a behavioural economics tool that is employed to influence the behaviour and decision-making of groups and individuals. For example, the ATO provides real-time messages to individuals completing their income tax returns if their work-related expenses are not within the expected range for their circumstances.
50 The ATO advised the ANAO on 22 July 2024 that other actions relate to payment receivables and non-lodgment risk models. Actions include: sending SMS messages; preventing self-service and requiring clients to call the ATO; recommending arrangements for paying debts; and issuing letters to ‘nudge’ clients.
51 The Data and Analytics Committee was responsible for ensuring an enterprise approach for data and analytics strategies and for providing strategic input and recommendations to other committees and decision makers. The Deputy Commissioner chaired the committee and membership included nine other Deputy Commissioners and the Chief Service Delivery Officer.
52 The Strategy Committee is co-chaired by the Second Commissioners of the Client Engagement Group and the Law Design and Practice Group. Its membership includes eight Deputy Commissioners from across the ATO.
53 Business line projects are those that do not meet the criteria of a corporate project and are planned and delivered with resourcing and funding from within the originating business line.
54 Section 15 of the Public Governance, Performance and Accountability Act 2013 requires that accountable authorities must govern their entities in way that promotes the: proper use and management of public resources; achievement of the purposes of the entity; and financial sustainability of the entity. This includes establishing decision-making processes, appropriate oversight and reporting arrangements.
55 Australian Government et al., National framework for the assurance of artificial intelligence in government, Australian Government, ‘Governance’.
56 See AS ISO/IEC 42001:2023 Information technology — Artificial intelligence — Management system, 16 February 2024, sections 5.1 and 5.3.
57 Smarter Data is a business line (or division) within the ATO. It is headed up by the Deputy Commissioner Smarter Data, a Senior Executive Service Band 2 officer.
58 Audit committees must review the appropriateness of an entity’s: financial reporting; performance reporting; system of risk oversight and management; and system of internal control. Section 17 of the Public Governance, Performance and Accountability Rule 2014.
59 ATO, Key committees, ATO, Canberra, 15 August 2023, available from https://www.ato.gov.au/about-ato/who-we-are/executive-and-governance/key-committees [accessed 27 June 2024].
60 The ANAO’s analysis focusses on the ATO Executive Committee and the Audit and Risk Committee due to their wide-ranging oversight of ATO operations, and on the Strategy Committee and Risk Committee due to their roles in relation to AI.
61 The Gen AI B2 Group is supported by the Generative AI SES Band 1 Working Group which is responsible for implementing and operationalising the ‘Use of publicly available generative AI technology policy’.
62 Digital Transformation Agency, Policy for the responsible use of AI in government, DTA, August 2024.
63 ibid.
64 Department of Industry, Science and Resources, Australia’s AI Ethics Principles, DISR 2019, available from https://www.industry.gov.au/publications/australias-artificial-intelligence-ethics-principles [accessed 11 November 2024].
65 Section 16 of the Public Governance, Performance and Accountability Act 2013.
66 Australian Government et al., National framework for the assurance of artificial intelligence in government, Australian Government, see ‘A risk-based approach’.
67 The Risk Management Chief Executive Instruction is a direction issued by the Commissioner of Taxation under the Public Governance, Performance and Accountability Act 2013.
68 A summary of these risks is also included in the ATO corporate plan. ATO, ATO corporate plan 2024–25.
69 Throughout this report, these risks are referred to as the ‘AI-related enterprise risks’.
70 ATO guidance states that controls are rated as ‘insufficient evidence’ if ‘controls are not yet functional or information is not available to determine the effectiveness of the controls’.
71 Shared risks include risks that extend across entities and potentially the community, industry, international partners and other jurisdictions. In large entities, shared risks can exist within the entity.
72 The ANAO’s Lessons product, Risk Management, identifies that ‘ANAO audits find that the management of shared risks is a particular area of weakness’, see ‘7. Consider additional factors for shared risks’, available from https://www.anao.gov.au/work/insights/risk-management [accessed 25 July 2024].
73 Department of Finance, Commonwealth Risk Management Policy, Finance, 29 November 2022, see Element Seven, available from https://www.finance.gov.au/government/comcover/risk-services/management/commonwealth-risk-management-policy [accessed from 22 July 2024].
74 Data and analytics includes: data activities; analytics activities; automation activities; and AI activities.
75 Department of Finance, Commonwealth Risk Management Policy, Element One.
76 Australian Government et al., National framework for the assurance of artificial intelligence in government, Australian Government, see ‘A risk-based approach’.
77 Organisation for Economic Co-operation and Development, Advancing Accountability in AI, OECD, 2023, p. 52, available from https://www.oecd.org/en/publications/2023/02/advancing-accountability-in-ai_753bf8c8.html [accessed 26 July 2024].
78 AS ISO/IEC 42001:2023 Information technology — Artificial intelligence — Management system states that an entity should establish an AI risk assessment — that establishes a process for identifying, analysing and evaluating risks — and an AI risk treatment process, p. 19.
79 In August 2024, the ATO combined the data ethics assessments and models ethics assessments.
80 AS ISO/IEC 42001:2023 Information technology — Artificial intelligence — Management system, p. 35.
81 The ATO defines a ‘data activity’ as: ‘any work that involves data throughout the data lifecycle, including strategy, acquisition, transformation, storage, access, analytics (including artificial intelligence, machine learning, and generative AI), automation, sharing, integration, archiving and disposal of data’.
82 The reliability and safety principle includes ‘ensuring that AI systems are reliable, accurate and reproducible, as appropriate’.
83 The accountability principle includes that ‘AI systems that have a significant impact on an individual’s rights should be accountable to external review’.
84 Australia’s AI Ethics Principles do not address legality as ‘the application of legal principles regarding the accountability for AI systems are still developing’. The European Union’s Ethics guidelines for trustworthy AI note that trustworthy AI should be ‘lawful’. European Commission, Ethics guidelines for trustworthy AI, Directorate-General for Communications Networks, Brussels, 2019, available from https://digital-strategy.ec.europa.eu/en/library/ethics-guidelines-trustworthy-ai [accessed 18 July 2024].
85 The data ethics team did not have dedicated resourcing until August 2023. Prior to this, other staff within the branch undertook data ethics work as part of broader roles.
86 The ‘Working in the ATO’ and ‘Safe, Secure and Inclusive’ training are mandatory for all new starters. Data Ethics Intermediate Training is required by all ATO officers who complete or review data ethics assessments.
87 ‘Privacy by design’ is a process for integrating good privacy practices into the design specifications of technologies, business practices and physical infrastructures. Office of the Australian Information Commissioner, Privacy by design, OAIC, n.d., available from https://www.oaic.gov.au/privacy/privacy-guidance-for-organisations-and-government-agencies/privacy-impact-assessments/privacy-by-design [accessed 29 July 2024].
88 ‘Secure by design’ is a proactive approach to the development of digital products and services that requires alignment of an organisation’s cyber security goals. Australian Signals Directorate, Secure-by-Design, ASD, n.d., available from https://www.cyber.gov.au/resources-business-and-government/governance-and-user-education/secure-by-design [accessed 29 July 2024].
89 One of the data ethics assessments was for a project that included 10 AI models. The ATO completed a data ethics threshold assessment and determined that a data ethics impact assessment was not needed.
90 At the time of the ANAO’s assessment, the ATO had not mandated the model ethics assessment process.
91 DISR, Australia’s AI Ethics Principles.
92 Australian Government et al., National framework for the assurance of artificial intelligence in government, Australian Government, see ‘5. Reliability and safety’.
93 Organisation for Economic Co-operation and Development, Advancing accountability in AI, OECD, 23 February 2023, p. 10, available from https://www.oecd.org/digital/advancing-accountability-in-ai-2448f04b-en.htm [accessed 19 June 2024].
94 The ATO’s IT Delivery Method is an umbrella term that incorporates three different IT project management methodologies: ATO Scaled Agile; ATO Delivery Method and the Business Developed Application Delivery Method.
95 The AS ISO/IEC 42001:2023 Information technology — Artificial intelligence — Management system, 16 February 2024 includes various references to establishing documentation requirements (7.5 and A.4.2), including for the design phase (B.6.2.3).
96 AI Ethics Principles should be integrated throughout the AI system lifecycle. Department of Industry, Science and Resources, Australia’s AI Ethics Principles, DISR, 2019, available from https://www.industry.gov.au/publications/australias-artificial-intelligence-ethics-framework/australias-ai-ethics-principles [accessed 17 July 2024].
97 Risk management must be embedded into the decision-making activities of an entity. Department of Finance, Commonwealth Risk Management Policy, Finance, 29 November 2022, available from https://www.finance.gov.au/government/comcover/risk-services/management/commonwealth-risk-management-policy [accessed 25 July 2024].
98 ‘Those responsible for the different phases of the AI system lifecycle should be identifiable and accountable for the outcomes of the AI systems’. Department of Industry, Science and Resources, Australia’s AI Ethics Principles.
99 Prior to December 2022, the ATO used on-premises infrastructure for these tasks.
100 Department of Home Affairs, PSPF Annual Release, available from https://www.protectivesecurity.gov.au/publications-library/pspf-annual-release [accessed 31 January 2025].
101 Section 2.2 of the Privacy Impact Assessment assesses the system’s compliance with each of the Australia Privacy Principles as set out in the Privacy Act 1988. Specifically, against Principle 11, the assessment is to rate the system as ‘complied’ based on the completion of penetration testing.
102 Department of Home Affairs, Protective Security Policy Framework, Policy 11: Robust ICT systems.
103 These reports are known as Service Organisation Controls (SOC) Reports and are conducted by third-party reviewers of the service provider’s environment.
104 There have been several changes to the ISM since this time.
105 ATO, Deductions for work expenses, ATO, 2023, available from https://www.ato.gov.au/individuals-and-families/income-deductions-offse… [accessed 24 April 2024].
106 Tax gaps estimate the difference between what the ATO expects to collect and the amount that would have been collected if every taxpayer was fully compliant with the law.
107 ATO, Latest estimates and trends, ATO, 2023, available from https://www.ato.gov.au/about-ato/research-and-statistics/in-detail/tax-gap/individuals-not-in-business-income-tax-gap/latest-estimates-and-trends [accessed 24 July 2024].
108 Organisation for Economic Co-operation and Development, ‘Toward algorithm auditing: managing legal, ethical and technological risks of AI, ML and associated algorithms’, OECD, May 2024, p. 7, available from https://royalsocietypublishing.org/doi/10.1098/rsos.230859 [accessed 27 September 2024].
Supreme Audit Institutions of Finland, Germany, the Netherlands, Norway and the UK, Auditing machine learning algorithms, Norway, 2023, available from https://www.auditingalgorithms.net/index.html [accessed 2 August 2024].
109 Department of Industry, Science and Resources, Australia’s AI Ethics Principles.
110 The AS ISO/IEC 42001:2023 Information technology — Artificial intelligence — Management system states that an organisation should ‘define and document the specific processes for the responsible design and development of the AI system’, paragraph A.6.1.2.
111 GitLab is a web-based repository for ‘Git’ (a commonly used version control system to track changes in computer files) and acts as a platform that allows officers to perform various tasks in a project.
112 Governments should maintain reliable data and information assets, ‘including records of decisions and testing, and the information and data assets used in an AI system’. Australian Government et al., National framework for the assurance of artificial intelligence in government, Australian Government, see ‘Transparency and explainability’.
113 The ANAO has previously made findings in relation to the ATO’s enterprise change management and segregation of duties. ANAO, Audits of the Financial Statements of Australian Government Entities for the Period Ended 30 June 2023, 14 December 2023, p. 374, available from https://www.anao.gov.au/work/financial-statement-audit/audits-the-financial-statements-australian-government-entities-the-period-ended-30-june-2023 [accessed 6 August 2024].
114 Department of Industry, Science and Resources, Australia’s AI Ethics Principles.
115 The National Institute of Standards and Technology, Towards a Standard for Identifying and Managing Bias in Artificial Intelligence, NIST, March 2022, p. 13, available from https://www.nist.gov/publications/towards-standard-identifying-and-managing-bias-artificial-intelligence [accessed 1 August 2024].
116 Australian Government et al., National framework for the assurance of artificial intelligence in government, Australian Government, see ‘Data governance’.
117 Supreme Audit Institutions of Finland, Germany, the Netherlands, Norway and the UK, Auditing machine learning algorithms, Norway, p. 31.
118 Government Accountability Office, Artificial Intelligence: An Accountability Framework for Federal Agencies and Other Entities, GAO, pp. 83–84, available from https://www.gao.gov/products/gao-21-519sp [accessed 1 August 2024].
119 AS ISO/IEC 42001:2023 Information technology — Artificial intelligence — Management system, 16 February 2024, Appendix B.6.2.5, pp. 33–34.
120 Supreme Audit Institutions of Finland, Germany, the Netherlands, Norway and the UK, Auditing machine learning algorithms, Norway, 2023, p. 36.
121 AS ISO/IEC 42001:2023 Information technology — Artificial intelligence — Management system, 16 February 2024, section 6.2.
122 Department of Industry, Science and Resources, Voluntary AI Safety Standard, DISR, 2024, p. 27, available from https://www.industry.gov.au/publications/voluntary-ai-safety-standard [accessed 18 September 2024].
123 Australian Centre for Evaluation, Evaluation toolkit: What is evaluation? and Why evaluate?, Treasury, available from https://evaluation.treasury.gov.au/toolkit/what-evaluation [accessed 8 May 2024].
124 AS ISO/IEC 42001:2023 Information technology — Artificial intelligence — Management system, 16 February 2024, sections 9.1 to 9.3.
125 AS ISO/IEC 42001:2023 Information technology — Artificial intelligence — Management system, 16 February 2024, section B.6.
126 Model drift is defined as changes in the relationship between the data inputs and the prediction outputs, and which can result in performance degradation, which may require retraining of the AI model.
127 Department of Industry, Science and Resources, Australia’s AI Ethics Principles, DISR, 2019, available from https://www.industry.gov.au/publications/australias-artificial-intelligence-ethics-framework/australias-ai-ethics-principles [accessed 8 August 2024].
128 Department of Industry, Science and Resources, Voluntary AI Safety Standard, DISR, Australia, 2024, p. 30.
129 Australian Government et al., National framework for the assurance of artificial intelligence in government, Australian Government, see ‘Accountability’, available from https://www.finance.gov.au/government/public-data/data-and-digital-ministers-meeting/national-framework-assurance-artificial-intelligence-government [accessed 9 August 2024].
130 Digital Transformation Agency, Policy for the responsible use of AI in government, DTA, August 2024, available from https://www.digital.gov.au/policy/ai/policy [accessed 16 August 2024].
131 AI management systems can include: policies and processes; governance frameworks; risk management; AI systems lifecycles; and monitoring and evaluation. AS ISO/IEC 42001:2023 Information technology — Artificial intelligence — Management system, 16 February 2024, section 10.1.
132 Digital Transformation Agency, Policy for the responsible use of AI in government, DTA, August 2024.
133 National Archives of Australia, Information management legislation, NAA, available from https://www.naa.gov.au/information-management/information-management-legislation [accessed 8 August 2024].
134 National Archives of Australia, Information management for current, emerging and critical technologies, NAA, available from https://www.naa.gov.au/information-management/managing-information-assets/types-information/information-management-current-emerging-and-critical-technologies [accessed 5 September 2024].
135 Australian Government et al., National framework for the assurance of artificial intelligence in government, Australian Government, ‘6. Transparency and explainability’.
136 As a result of the ATO’s poor information management in relation to the adoption of AI, the ANAO used an information collection methodology which involved the extraction of mailboxes of relevant ATO officials.